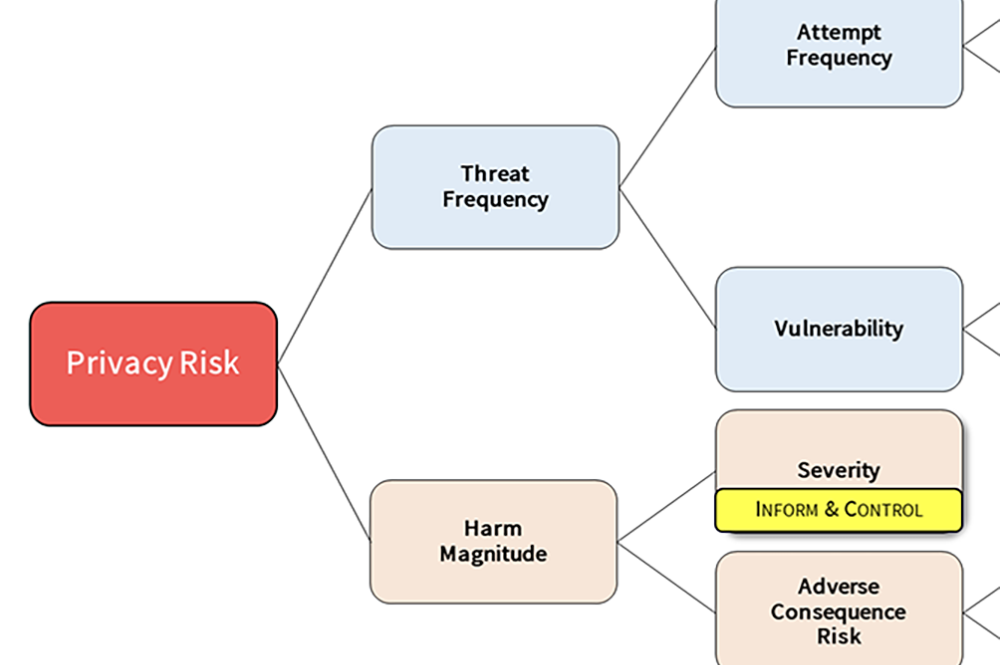
When I saw Jack Jones present on FAIR™ at an IANS Research Forum several years ago, it was like a light bulb went off in my head. I immediately ordered the FAIR book and began a cover-to-cover reading, twice. I had been unsatisfied with existing methods to assess privacy risks and I was excited to apply my new-found knowledge of FAIR to privacy.
What follows is an introduction to that application. I don’t have the space to do a complete breakdown, but, assuming that most readers are familiar with FAIR in the information risk context, I’m going to highlight some key differences as to how I apply a modified version in a privacy context.
A more thorough breakdown can be found in the IEEE paper Quantitative Privacy Risk by myself and Stuart Shapiro, a copy of which is also available on my website, and in the 2nd Edition of my book, Strategic Privacy by Design (IAPP 2022).
An Excel-based risk calculator applying FAIR to privacy can be found at the NIST Privacy Engineering Collaboration space.
Privacy is not about the security of personal information
There is a misperception by many that “privacy” is merely about the security, or confidentiality, of personal information. Privacy is a much broader concept than security. While I have many examples, I’ll use one that tends to resonate with people.
A few years ago, when Pokémon Go came out there was concern about what information it was collecting about players. But there was another privacy invasion perpetuated by the game that had nothing to do with player data.
In the game, players were encouraged to visit churches (physical real-world locations) and "train" their characters. Unfortunately, some of the churches identified by the game had actually been purchased by individuals and converted to their private residences. These home-owners now had Pokémon Go players showing up at odd hours outside their houses to play the game.
This is an example of intrusion, a violation of the homeowner’s tranquility or solitude, the peaceful enjoyment of their residence. It is not an information privacy violation, but an invasion nonetheless. It’s the same sort of privacy violation one feels when spam hits your email, a telephone solicitor calls while you’re eating dinner or sidewalk vendors bark at you when you’re on your way to lunch.
Any notion of privacy risk must capture these types of non-information privacy invasions in addition to those involving insecure personal information.
There are several different normative models for classifying privacy harms, but I’m partial to Dan Solove’s Taxonomy of Privacy which identifies 15 types of privacy harms, in four categories: information processing, information dissemination, collection and invasions (like Pokémon Go).
Threat actors not threat actor communities
FAIR uses the concept of threat actor communities to group actors as those who might pose a risk. This works great when looking at how unauthorized actors might pose risks to an organization. Even internal actors, such as employees, may pose risk when acting in an unauthorized manner, such as selling data on the black market.
But when looking at privacy risk, oftentimes individual actors in an ecosystem create risk. Employing a web host, for instance, may introduce additional privacy risk over self-hosting a website. You could lump all vendors together but because they perform different functions within a system, the analysis should be particularized.
Consider the ecosystem around a mobile health care application. You have the developer of the application, a hosting provider, the network provider, the operating system on the phone, the healthcare provider, etc.
You wouldn’t consider developers as a community of threat actors, but the particular developer who created and managed the application might be. That particular developer has affordances, by virtue of being the developer, that the broader community of developers don’t. This doesn’t mean that some threat actors aren’t part of a broader community. You still have hackers, insurance companies, and others that, while acting individually, pose the same sorts of risks as any other hacker, insurance company or other.
Privacy risk is individual risk not organizational
Perhaps the biggest distinguishing factor is my view of looking at risks to individuals not organizations (in terms of fines, lawsuits, reputational damage, etc.). In fact, the General Data Protection Regulation (Article 25(1)) requires that organizations look at “risks of varying likelihood and severity for rights and freedoms of natural persons” when designing products and services--not the risks to the organization. Similarly, the NIST Privacy Framework, defines privacy risk as “[t]he likelihood that individuals will experience problems resulting from data processing, and the impact should they occur.”
There are several reasons for this. First, privacy violations are often externalities of organizational activities, with cost borne by individuals while the benefits accrue to the organization. The situation analogizes to pollution, where the polluter doesn’t pay the full social cost imposed by their activity. A clear-cut privacy example would be use of information about consumers for price discrimination. If an organization uses the fact that a person uses an iPhone to charge higher prices, the benefit (higher earnings) accrues to the organization and the costs (paying more) fall to the individual.
Secondly, I would submit that an organization’s focus on risk to itself creates a perverse incentive to reduce identification of privacy violations rather than actually alter behavior to reduce privacy invasions of individuals. Consider a hotel chain that wants to put surveillance cameras in their rooms.
Clearly there is an organizational risk associated with this violation of guests’ privacy. Guests finding out might quit going to the hotel, they might sue, or criminal charges could be filed against hotel management. Reputation damage would be potentially devastating.
Many of the controls the management could consider would reduce “privacy risk” but if the measure is organizational risk those controls reduce the likelihood that the activity is uncovered. They could make the cameras smaller (“pinhole cameras”), they could encrypt the channel to prevent others from finding the transmission, etc. I’m reminded of the movie The Imitation Game where the British code breakers decided not to take action based on decrypted communications because it could alert the Germans their code was broken. Similarly, the hotel might not call the police if they see someone stealing the towels because that would alert authorities to their cameras. They might, instead, develop a plausible parallel construction to their knowledge that someone stole towels, like insuring the cleaning staff counted towels in that room
All of these reduce risks to the organization from civil and criminal liability but none of it reduces the violative nature of their activity, namely the surveillance of their guests. Organizations actually do this all the time. They often obfuscate their activities in their privacy notices, burying them in legalese, so as to be “transparent” but not really informing customers of the extent of what they do lest those customers leave to less invasive competitors (an organizational risk of reputational damage of their privacy invasions).
Mapping risk factors to controls
One of my biggest epiphanies when applying FAIR to privacy was that the factors mapped so perfectly to the control strategies that I use, a set of eight privacy-by-design strategies from Jaap-Henk Hoepman:
Opportunity (contact frequency in FAIR) can be reduced by Minimizing information or Separating information amongst potential threat actors.
Motivation (probability of action in FAIR) can be reduced by Enforcing policies against threat actors or making them Demonstrate compliance with policies.
Difficulty (resistance strength) can be reduced by Hiding information (think encryption, access restrictions, etc.) from threat actors or Abstracting data (reducing granularity) they do have access to.
Finally, reducing the severity, (in other words turning activities from violations to non-violations) can be done through Informing individuals or giving them Control. Think of it like this, the hotel, in the above example, could tell guests about the in-room camera and give them the option of turning the camera on or off. If the guests know about it and have control over it, the camera no longer becomes a violation, but potentially becomes a selling feature (for instance for an elderly guest that is concerned about injury and being unable to call for help).
The control strategies to risk factors mapping is not meant to be exclusive. Some controls will also indirectly affect the factors. Informing customers about the hotel cameras will lead to many of them turning off the cameras (reducing opportunity). Encrypting (hiding) the camera communication link will reduce motivation of some threat actors because they have to expend effort to bypass the encryption.
While this doesn’t represent the entirety of how I’m applying FAIR to privacy risk, I hope it provides some interesting concepts for others to think about. I welcome comments, feedback and questions.
.
About the author: R. Jason Cronk is the author of Strategic Privacy by Design, a licensed attorney in Florida, designated a Fellow of Information Privacy by the International Association of Privacy Professionals and a Privacy by Design Ambassador by the Information and Privacy Commissioner of Ontario, Canada. He is also the chair of the newly formed Institute of Operational Privacy Design, a non-profit promoting a standard around privacy design. He consults and conducts training through his firm Enterprivacy Consulting Group and can be found tweeting at @privacymaverick