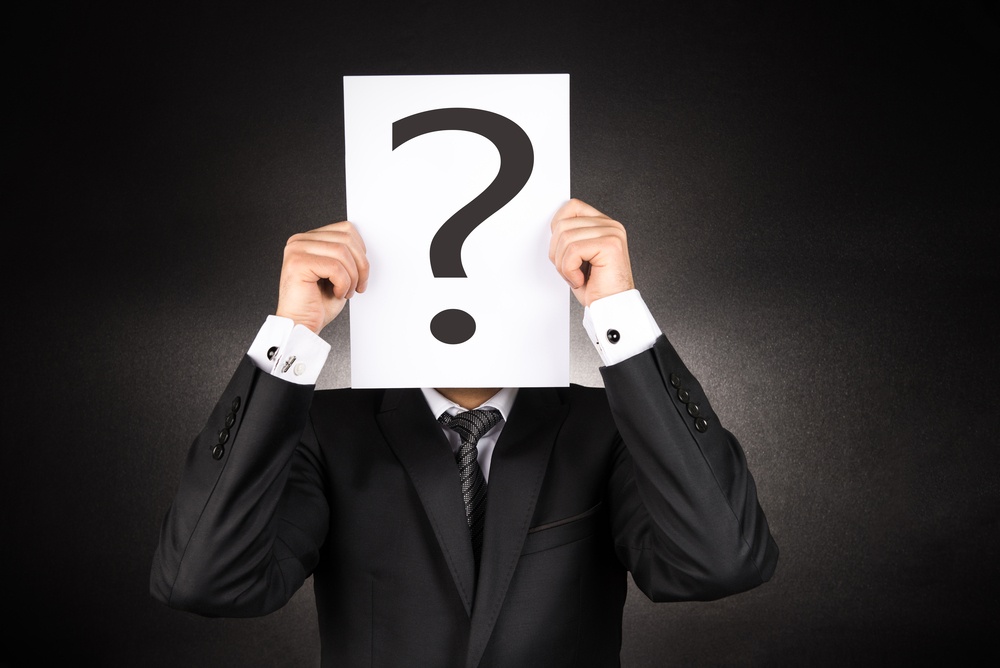
There was a question recently on the FAIR Institute Members LinkedIn forum regarding “unknowns”, specifically, “How do we analyze the risk of not knowing what threats and vulnerabilities we might not be aware of that could lead to losses?” There are a couple of ways to interpret this question:
- How do we account for the unknowns in a single analysis?
- More strategically, how do we evaluate the risk an organization faces from incomplete visibility into its risk landscape?
Both of these are important questions that deserve a useful answer. In order to keep these posts a bit shorter, I’ll answer the first question in this post, and the second question in a subsequent post.
Understanding the problem(s)
There are a couple of immutable facts regarding risk analysis:
- There are always unknowns
- #1 above is true whether you’re doing qualitative or quantitative risk measurement
You are never likely to have perfect visibility into the assets within a risk scenario, the threats against those assets, or the condition of controls meant to protect the assets. Furthermore, even if you did have perfect information into what has transpired in the past, the future rarely unfolds exactly like the past. And risk analysis is almost always about the future.
The fact that qualitative measurements are more subjective and inherently imprecise, doesn’t alter the fact that these unknowns exist. Those characteristics of qualitative analysis simply make it easier to avoid dealing with unknowns. Sweep them under the rug, so to speak. When doing quantitative analysis, however, you are forced to face the uncomfortable truth. After all, how do you put numbers around something you don’t have complete information for?
Solutions to the problem(s)
Douglas Hubbard’s book, How to Measure Anything is a great resource for learning how to deal with imperfect information in an analysis. If you’re serious about doing risk analysis, you (at least) need to read his chapter on calibrated estimates. Short of that, simply keep in mind that ranges are your friend.
For example, every time I teach a course in risk analysis I will point to someone in the class, and ask them to estimate how tall I am. The answers I’m given are always precise — e.g., 5’11”. Whenever I ask the person who made the estimate whether they would bet $1000 of their own money on their estimate, the answer is an emphatic “No!”. When I ask whether they can give me a range that they would bet $1000 on, they invariably say “Of course!” and then give a range like, “Between 5’3” and 6’3”.” In these instances, the person making the estimate is accounting for their imperfect information by creating a range that’s wide enough for them to bet money against.
There is absolutely nothing wrong with using ranges as measurement values. In fact, ranges are a great way to express measurement uncertainty — i.e., the wider the range, the greater the uncertainty. This is important information that enables decision-makers to better interpret and use analysis results.
Another critical part of the solution is to be very clear on what is in and out of scope for an analysis. For example, let’s say I’m doing an analysis to measure the risk associated with databases in the environment. In this instance I will very clearly call out the fact that the scope for this analysis does NOT include databases that are not centrally managed (You know, the ones on servers under people’s desks or being managed by shadow IT groups.). If I were to try and include those with the centrally managed database analysis, the higher degree of uncertainty would require that I use wider, flatter distributions for input values. The result would be less precision in the output. The output also would be less actionable. If I wanted to analyze those rogue databases, I’ll do a separate analysis with input values that reflect much less certainty. Parsing this into two separate analyses also can highlight the difference in risk levels and uncertainty between the two populations of databases, which can be extremely useful when communicating the value of centralized management.
As for historical data’s relevance to future conditions, the trick is to take trends into consideration. Have threat events been increasing within the scenario being analyzed? Have they been decreasing? Staying steady? With regard to control conditions, are processes improving and thus we’d expect higher levels of compliance? Or have new controls been put into place that are expected to increase efficacy? Conversely, have controls remained the same but more and more exploits been coming out for the technology under analysis? There is no magic bullet here — you have to evaluate the past, leverage the best information you have about how things are trending, and then make an estimate that reflects that analysis. Here again, this should be true regardless of whether you’re doing a quantitative or qualitative analysis.
The bottom line
It’s almost (almost, but not really) funny that nobody seems to sweat uncertainty and unknowns when they do typical wet finger in the air risk ratings. All of a sudden though, they get very nervous about unknowns when faced with doing a more rigorous analysis. Yet those same unknowns exist nonetheless. One of the advantages to quantitative analysis is that it forces the analyst to recognize and deal with these unknowns, and in doing so almost certainly come up with a more accurate and useful result than if they ignore unknowns altogether.
In the next post I'll discuss the more strategic question related to unknowns. Stay tuned...