Using the FAIR Model as the ‘Swiss Army Knife’ of Privacy Uncertainty Quantification for GDPR
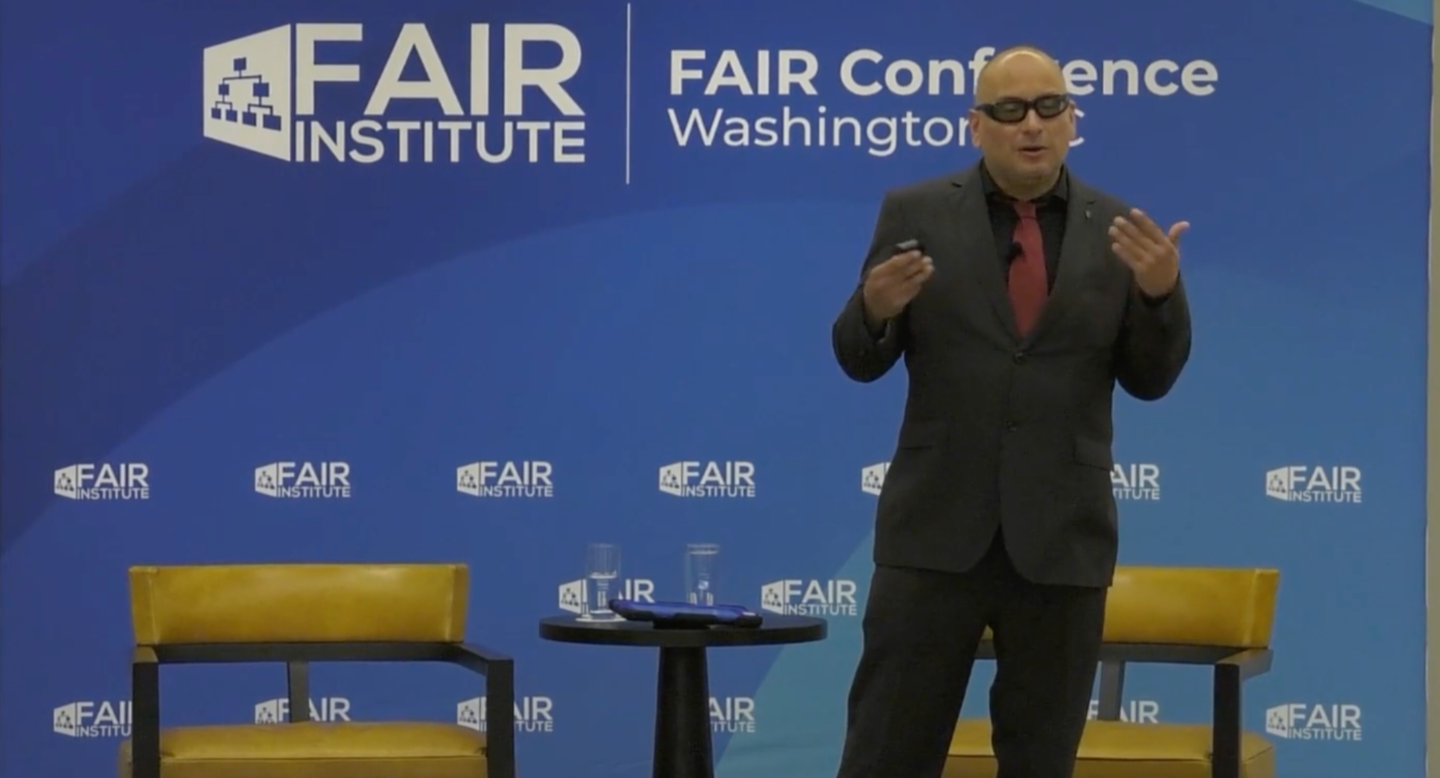
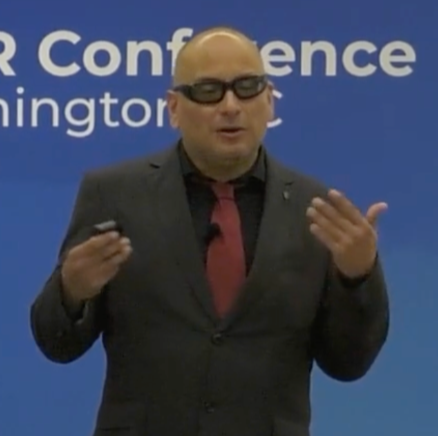
Privacy uncertainty quantification for GDPR compliance is still a largely unknown term, but a very promising one. It can be justified from two perspectives. From a data subject’s point of view, it can be understood as the need to reduce uncertainty for better protection of the rights and freedoms of the data subjects.
FAIR Institute Member Luis Enríquez is Professor at the University of Lille (France) and General Director of Data Security at the Superintendencia de Protección de Datos Personales (Ecuador)
He spoke at the 2023 FAIR Conference on Using the FAIR Model for AI Risk-Based Accountability.
In simple terms, a personal data breach would provoke material losses due to the violation of other fundamental rights, such as the right of non-discrimination. From a data controller’s point of view, it can be understood as the need to reduce uncertainty for complying with data protection/privacy legal regulations, where the lack of compliance can trigger an administrative fine.
The current state of the art of privacy/data protection risk management is very superficial, and the sad truth is that privacy impact assessments are still practiced as checklists.
This superficial state of the art may be justified based on two problems of measuring privacy: Firstly, people value their own privacy differently. Secondly, there are special vulnerable groups of data subjects that may suffer a higher impact in a data breach risk scenario.
Yet, judges and administrative authorities still need to quantify a violation of the rights and freedoms of the data subjects, by exercising their enforcement powers.
The Personal Data Value at Risk (Pd-VaR) Approach
To address these difficulties, I created a Personal Data Value at Risk (Pd-VaR) approach. In a nutshell, The Pd-VaR approach considers that Data Protection Officers (DPOs) and Chief Information Security Officers (CISOs) are not judges or administrative authorities, so they don’t have the training and the competence to forecast an impact on the rights and freedoms of natural persons.
So, how can they estimate the impact of a data breach on the rights of the data subjects? My solution was to use jurimetrics (the scientific study of the legal system) and data protection predictive analytics to implement information and argument retrieval models from historical data taken from existing administrative fines. That helped me to profile the sanctioning psychology of the regulators and to obtain data from their legal decision-making arguments. This first task was called the Jurimetrical Pd-VaR.
The second task was to merge such profiling data with the current state of risk-based compliance maturity of a data controller, which I called the Calibrated Pd-VaR. Consequently, I was looking for a risk model that allows merging information security risks and data protection compliance risks.
Adapting the FAIR Model to Data Protection Compliance Risk
My first implementations were based on the FAIR model, as it divides the Loss Magnitude (LM) into primary losses and secondary losses. In a data security breach scenario, data protection administrative fines are, indeed, secondary losses.
This worked fine, but I still needed to model personal data protection losses to get the Secondary Loss Event Frequency (SLEF) and the Secondary Loss Magnitude (SLM).
I thought that using the same FAIR ontology for calibrating the SLEF and the SLM may provide me with an independent data protection risk calibration outcome, before adding its values into my main FAIR model cybersecurity risk scenarios.
I used the FAIR ontology but replaced the definitions behind the model branches:
This FAIR adaptation for GDPR risk-based compliance was the beginning of my FAIR ‘Swiss Army knife’ adventure. In the Loss Event Frequency (LEF) dimension, I could use the Threat Event Frequency (TEF) branches for my jurimetrical Pd-VaR outcomes, and the Vulnerability branch for my calibrated Pd-VaR ones.
In the Loss Magnitude (LM) dimension, as the risk scenario was only compliance risk, the GDPR administrative fine is usually considered the primary loss, and reputational losses are common secondary losses.
I used this approach for two risk-scenario approaches:
First, for cybersecurity risk scenarios, it was convenient to add the outcomes of the LEF and the Loss Magnitude (LM) separately. For the LEF outcomes, it was about determining the right percentage that could represent an administrative fine due to data breaches. For the LM, the outcomes could become the rationales of the ‘fines and judgments’ FAIR model’s branch.
Secondly, the derived model was also useful in pure GDPR compliance with only legal obligations, such as the lawfulness of processing.
After realizing this risk-based ‘Swiss Army knife’ nature of the FAIR model ontology, I implemented it for the complicated task of measuring the impact of a privacy risk on the rights and freedoms of the data subjects.
To accomplish this, I used statistics and probabilistic methods to calibrate the vulnerabilities of groups of data subjects against a risk scenario, with vulnerable groups such as elderly people, handicapped people, and so on.
I adapted the ontology to the needed branch definitions, where the key to calibrate such data subjects’ vulnerabilities was the vulnerability branch:
These data subject impact models can certainly help Data Protection Authorities to enhance their legal decision-making processes. Likewise, they can help as a reference to data controllers and processors while doing their Data Protection Impact Assessments. ‘
I keep using the FAIR model ontology as the ‘Swiss Army knife’ in other domains, particularly in the fundamental rights impact assessment established in the recent Artificial Intelligence Act, or as Algorithm Impact Assessments mainly in AI hallucination and adversarial machine learning risk scenarios.
My conclusion is that even though there is only one original FAIR model, the FAIR model ontology can be adapted as a ‘Swiss Army knife’ for many risk scenarios beyond cybersecurity. I strongly believe we can make data protection/privacy risk management better with a FAIR approach to privacy/data protection risk-based compliance. So, let’s keep researching.