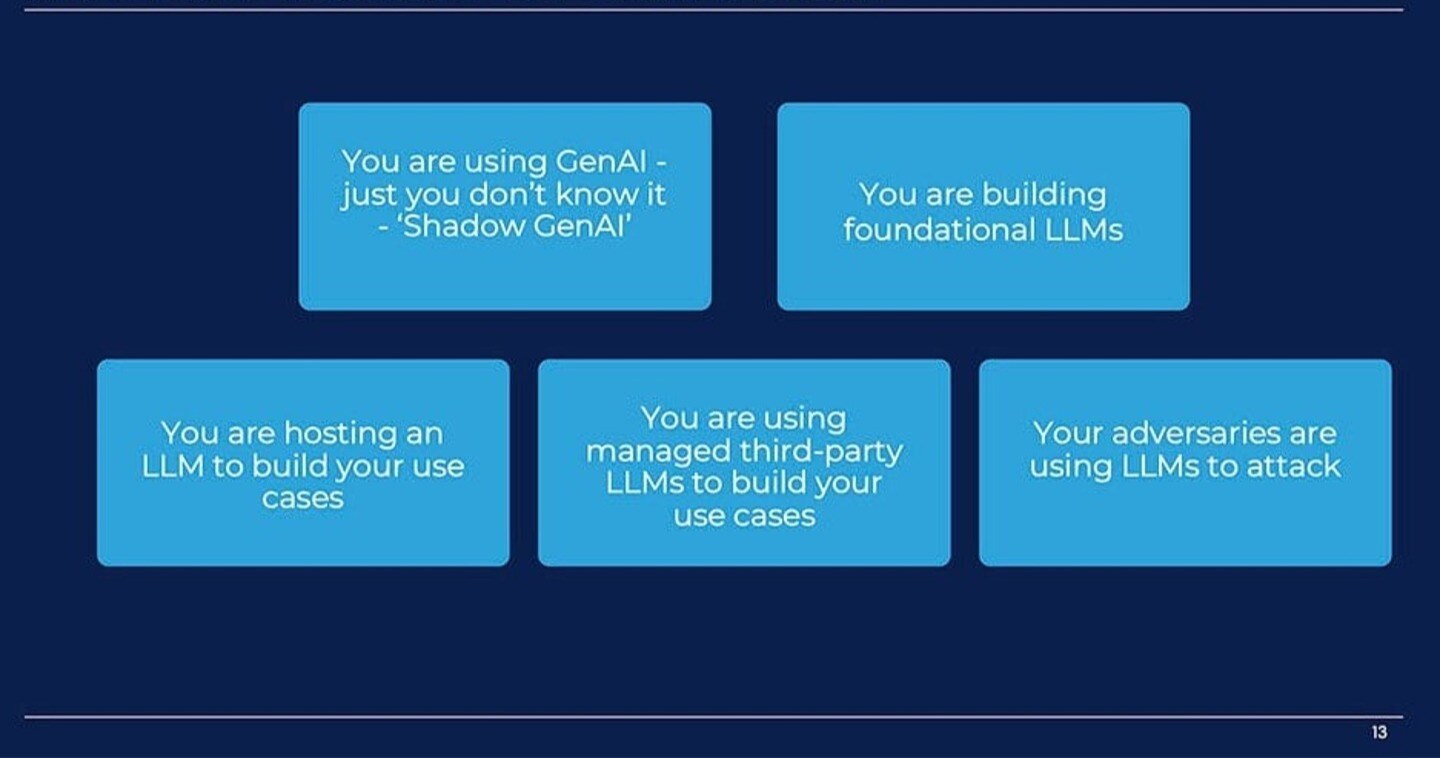
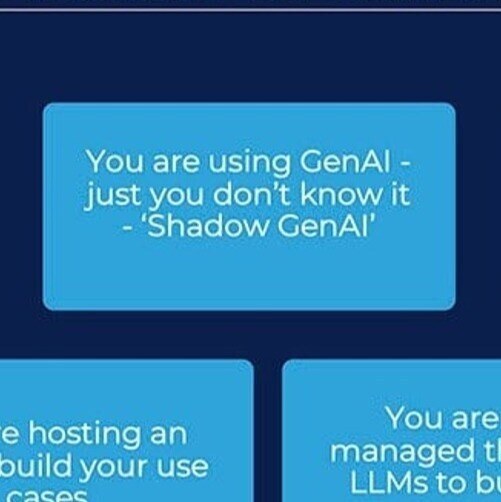
Join us at the 2024 FAIR Conference in Washington, DC (training September 29-30, sessions October 1-2), to explore the challenging new world of risk analysis for artificial intelligence.
The good news is we can apply our trusty techniques of FAIR (Factor Analysis of Information Risk) to do quantitative analysis for AI, just with new threat vectors, new risk scenarios, new loss categories – in other words, it’s the same but completely different.
FAIR Conference 2024 Will Present these AI-Related Events:
Workshop: Mastering AI Governance and Risk Management
1:00-5:00 PM, Monday, September 30
Leaders from the FAIR Institute AI Workgroup:
>>Jacqueline Lebo, Risk Advisory Manager, Safe Security
>>Arun Pamulapati, Sr. Staff Security Field Engineer, Databricks
Panel Discussion: Accelerating AI - Achieving the Right Balance Between Speed and Security
11:15 AM - 12:00 PM, Wednesday, October 2
Moderator:
>>Pankaj Goyal, Director, Research and Standards, FAIR Institute
Speakers:
>>Randy Herold, CISO, ManpowerGroup
Oki Mek, CISO, U.S. Federal Civilian Government, Microsoft
Michelle Griffith, VP, Security GRC, IHG
FAIR Inst. Workgroup Presentation: Navigating the Complexities of Assessing and Managing AI Risk
1:30-2:10 PM, Wednesday, October 2,
Moderator:
Omar Khawaja, CISO, Databricks
Speakers:
Arun Palmumati, Sr. Staff Field Engineer, Databricks
Jacqueline Lebo, Risk Advisory Manager, Safe Security
Register for the 2024 FAIR Conference!
FAIR Conference 2024 AI Workshop Preview
Consider starting your FAIRCON AI journey with the pre-conference workshop. Here’s a brief preview of just some of the topics the workshop will cover:
5 Steps to Quantification: Introducing FAIR-CAM
The FAIR Institute’s Artificial Intelligence Workgroup is doing truly pioneering work on AI-related risk, and has produced the
Using a FAIR-Based Risk Approach to Expedite AI Adoption at Your Organization
a great starting point for understanding the risk dimensions of AI, with the discipline of FAIR.
The workshop will introduce the playbook and walk you through its 5-step approach:
5 Threat Vectors for Artificial Intelligence
Here’s an example of how cyber risk analysis must adapt to the new architecture of large language models (LLMs) and generative AI (Gen AI): Only #1 vector will sound familiar, an external attack, while the others could be seen as new forms of insider or third-party risk.
- Active Cyber Attack: Your adversaries are using LLMs to attack you
- Shadow GenAI: You are using Generative AI, and you just don't know it.
- Foundational LLM: You are building LLM(s) for use cases.
- Hosting on LLMs: You are hosting an LLM and using it to develop use cases.
- Managed LLMs: You are using a third party LLM to develop use cases
5 New Gen AI Scenarios
Risk scenarios are the raw material of FAIR analyses. The workshop will go over these new forms of scenarios, and the challenges of quantification. For instance, what’s the bottom-line impact of a hallucination?
- Prompt injection: A direct prompt injection occurs when a user injects text that is intended to alter the behavior of a large language model (LLM).
- Model theft: Steal a system’s knowledge through direct observation of their input and output, akin to reverse engineering.
- Data leakage: AI models leak data used to train the model and/or disclose information meant to be kept confidential.
- Hallucinations: Inadvertently generate incorrect, misleading or factually false outputs, or leak sensitive data.
- Insider incidents related to GenAI: For instance, insiders using unmanaged LLM models with sensitive data.
7 Questions to Ask before Onboarding an AI system
AI-powered applications are on their way to being commonplace, both customer-facing and for internal use; the workshop will discuss the pros and cons of various ways to implement.
- Will we implement this solution?
- Will we need additional cyber insurance?
- Will we need additional controls?
- Should we buy an enterprise Generative AI solution?
- Should we use a third-party solution, or should we use a homegrown solution?
- Should we launch this app or alter safety rates for inputs in testing more?
- Does this model meet our risk tolerance, or should we use another one?