How to Get Started with FAIR Analysis for GenAI Risk
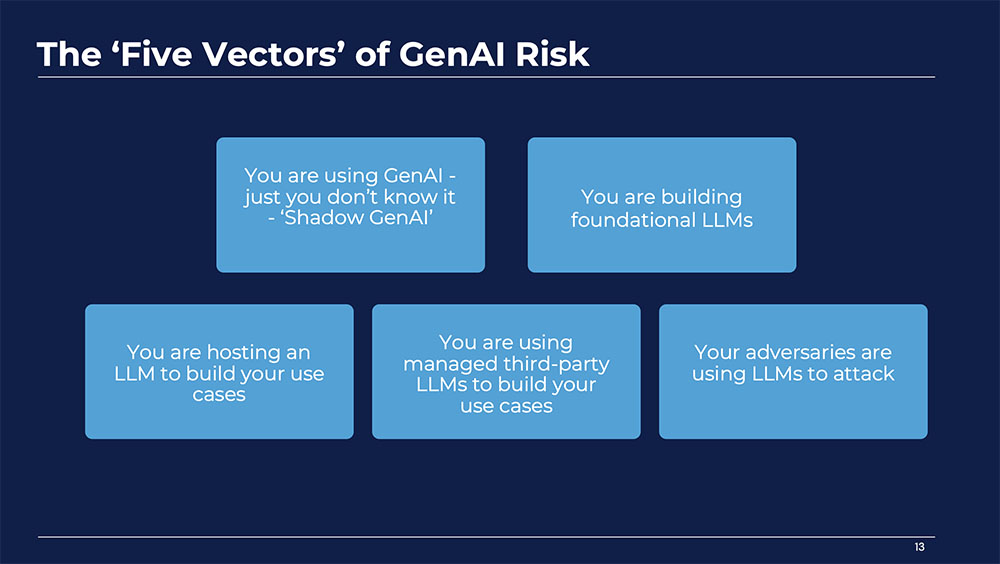
If you’re looking to understand the problem space for generative AI risk management and take first steps to apply FAIR quantitative risk analysis to GenAI, this video session from the recent FAIR Conference (FAIRCON23) is the place to start.
Accelerating Your GenAI Adoption through AI Risk Posture Management
Presenters:
Brandon Sloane, Information Security Risk Management Lead, AI GRC, Meta
Pankaj Goyal, Director, Standards & Research, FAIR Institute
A free FAIR Institute membership required to view. Join now.
A few of the many takeaways on AI risk from this session:
Your artificial intelligence risk use cases will come from five sources
- “Shadow GenAI” – your organization uses it without your knowledge
- You are building foundational large language models (LLMs) internally
- You host your own LLM
- A third-party hosts your LLM
- Attackers use LLMs against you
The two most likely decisions on GenAI you will have to support with risk analysis:
- Should we host an LLM internally? or
- Should we use a third-party hosted LLM?
Brandon: “A great way to think about this is the same way we approached cloud years ago…Think about what it means when you leverage somebody else’s service. What are you giving up and what are you gaining?”
Two likely AI risk scenarios – and FAIR analysis problems to solve
Pankaj and Brandon tackled two scenarios you may well run into:
>>Scenario 1 – Disclosure of private, confidential or sensitive information
They focused here on estimating the Susceptibility factor in FAIR modeling, dependent on strength of controls. Pankaj demonstrated research work done by Safe Security and the FAIR Institute and shown on GenAIRisk.ai: a library of controls relevant to AI and mapped to the FAIR Controls Analytics Model (FAIR-CAM™), a scoring of vendors of third-party SaaS services for AI based on their security features, and a library of GenAI risk scenarios.
>>Scenario 2 – Regulatory or user harms due to generation of undesirable content
They discussed the challenge of defining Loss Event Frequency in FAIR analysis. “The way we have defined ‘loss event’, it’s been very clear that a loss event has occurred,” said Brandon. But take the example of an AI generating a joke that’s offensive by some definitions: “Is this a loss event to every person who sees it?...This is an open question for the FAIR community.”
Operationalizing risk scenarios: How risk managers can support business decision-making on artificial intelligence
FAIR quantitative risk analysis could help at each phase of development of an AI product. Pankaj and Brandon outlined the process, starting from
- Define requirements (such as host or outsource an LLM) to
- Deploy (such as making API calls – a new source of risk)
But “nothing different here – we’ve been doing this for years,” Brandon said. “The only challenge is, what are the AI risks that you haven’t had to deal with before and are there additional controls and costs for standing these things up to bring us to an acceptable risk?
Watch the Video on Demand Now: Accelerating Your GenAI Adoption through AI Risk Posture Management
A free FAIR Institute membership required to view. Join now.
Also from FAIRCON23:
Good News on AI Risk: We Can Analyze It with FAIR