Jack Jones Releases New Edition of the CRQ Buyer’s Guide to Cut through Risk Quantification Hype (Q&A with Jack)
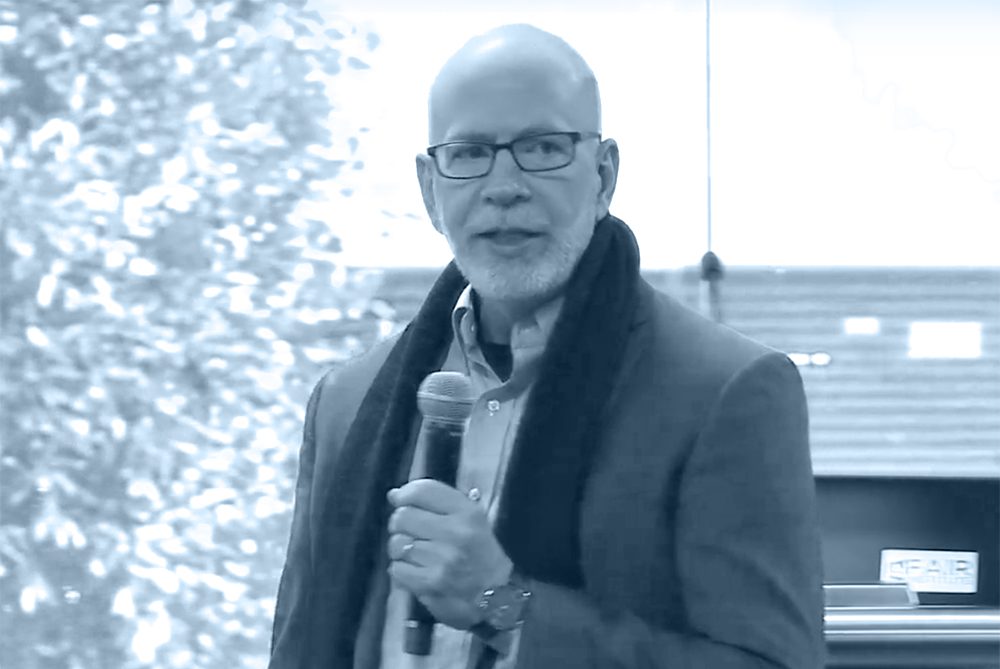
Looking to learn if cyber risk quantification (CRQ) is a good fit for your organization, then choose a CRQ solution? Well, good luck. The marketplace has been rapidly filling up with vendors loudly claiming that they provide CRQ. Jack Jones, creator of Factor Analysis of Information Risk (FAIR™), the standard for cyber risk quantitative analysis, has updated his buyer guide to give you a solid understanding of CRQ and separate hype from reality.
Download now: Understanding Cyber Risk Quantification: A Buyer’s Guide
FAIR Institute Contributing Membership required to download. Join now!
In this extensive guide, Jack covers:
>>The definition of CRQ and its value
>>Common concerns about adoption
>>The risk-measurement techniques you shouldn’t confuse with CRQ
>>Questions to ask a CRQ vendor
>>The red flags to warn you off a vendor
Jack answered some questions about the new Buyer’s Guide and the state of the CRQ marketplace:
What motivated you to update the Buyer’s Guide now?
Lots of players are entering the cyber risk quantification market, so it’s even more important now to make the marketplace aware of the distinctions on what qualifies as CRQ and what qualifies as better vs. dangerous CRQ. It’s really an attempt to help the market make good decisions about the solutions that they purchase.
What are the main misconceptions about CRQ?
There are several common misconceptions:
1. One of the most common misconceptions is that an organization must have some theoretical minimum amount of data to quantify risk. Yes, more data can reduce uncertainty (provided it’s the right data and applied correctly), but the misconception implies two things that aren’t true:
>>That qualitative risk measurements aren’t also data-dependent. The truth is that when someone waves their wet finger in the air and chooses a qualitative value, they are 100% of the time applying data. They’d have to be, otherwise what would drive a choice of “medium” versus “high”? Unfortunately, in qualitative analyses, the data being used are undefined and can’t be challenged or calibrated.
>>That you can’t get meaningful quantitative risk measurements with sparse data. Douglas Hubbard has done a great job of describing why this is untrue in his books and presentations (which in my opinion should be required reading in our industry).
2. The misconception promoted by many vendors is that it’s just a matter of plugging in a solution, you don’t have to know what’s going on underneath it. Simply trust in their algorithms and AI. After all, what could possibly go wrong? I make the analogy to encryption: Nobody buys proprietary encryption because there are so many ways it can be broken. I will argue tooth and nail that it's at least as easy to screw up cyber risk quantification, and that solutions need to be open so the community can inspect, understand, and challenge them.
3. That it’s only the numbers a CRQ analysis spits out that matter. If you talk to anybody who has used FAIR, almost invariably they will say that one of the most valuable contributions of the analysis process is the conversation it engenders – a mutual understanding amongst analysts and stakeholders of the problem being addressed, as well as the assumptions involved, the data being applied, and what the results mean. That shared understanding is so valuable, it can’t be overstated.
Join Jack Jones for a webinar on Understanding Cyber Risk Quantification.
Thursday, March 30, 2023, at 11 AM ET
Isn’t CRQ also perceived as difficult?
I get why people are averse to complexity, but if the medical profession had insisted on maintaining simplicity, bloodletting would still be “best practice”. Yes, we need to be pragmatic, but knowing where and how to legitimately simplify something requires first that we understand the complexities. Unfortunately, the CRQ market is so nascent and uninformed that they insist on unrealistic levels of simplicity and focus on dashboards with pretty colors and numbers. Too often, people don’t stop to examine how those numbers are arrived at or whether they are accurate.
If qualitative or bad quantitative approaches don’t work, how do they persist?
Fortunately for most organizations, significant loss events are rare. Consequently, it’s easy to presume that organizations are prioritizing well and choosing good solutions – which are the outcomes driven by risk analysis and measurement. But when significant loss events do occur it doesn’t take rocket science to recognize that a lot of things that should have been addressed weren’t. Also, if you examine many of the “risk measurements” organizations do, they are embarrassingly bad. Furthermore, many CISOs will admit that their organizations have one (or more) of almost every conceivable security technology. They’ll also usually admit that much of their security technology either isn’t being used, or at least isn’t being used effectively.
What this tells us is that prioritization and solution selection is not working well in the vast majority of organizations. At least, if by “working” we mean that priorities are appropriate and solutions are providing good risk reduction value. The bottom line is that we shouldn’t confuse “haven’t had a big breach yet” with “we’re measuring risk well, and prioritizing and applying our resources effectively.”
You’re an advocate for the FAIR model. But what’s the importance of a model for CRQ?
Any measurement that involves more than one parameter requires a model. When you have something as profoundly complex as cybersecurity, you absolutely need a well-defined model to have much hope of reliability and consistency.
Following cybersecurity controls frameworks and related maturity models is a standard approach to risk management. You’ve argued that these frameworks only take you so far, and you recently introduced the FAIR Controls Analytics Model (FAIR-CAM™) to complete the picture.
Current frameworks describe the components that can or should make up a security program – i.e., security program anatomy. They do not describe how these components affect risk. For instance, if we improve our logging, how does that reduce risk? Does it reduce frequency or magnitude or both? And in what circumstances does it reduce risk? Is it dependent on other things to have any effect at all? These things are not defined in the existing frameworks. We have to understand how controls affect risk directly or indirectly – what I refer to as controls physiology – and that’s what FAIR-CAM provides.
How is the trend toward adoption of CRQ looking to you? Are you optimistic?
I am optimistic but it’s a marathon not a sprint. Boards are beginning to want better information about ROI on cybersecurity investment, regulators are slowly beginning to push in this direction, organizations like the World Economic Forum and the National Association of Corporate Directors are saying you absolutely must do quantification for your program to be mature. All are helping to move the profession forward.
But there is still resistance. No profession likes to change in fundamental ways, and this is one of the reasons why people gravitate to over-simplified solutions. Just plug in this platform and you can say you do CRQ and go back to what you were doing before.
In the long run, I see no reasonable alternative to eventual adoption of these methods because logically it’s the only way organizations can hope to apply their limited resources effectively, and significantly improve their odds of winning against the bad actors. And eventually, organizations will get tired of pouring money down a dark hole based on practices that fundamentally don’t work. The future is quantitative. It’s just a question of how long it will take.
Download now: Understanding Cyber Risk Quantification: A Buyer’s Guide
FAIR Institute Contributing Membership required to download. Join now!