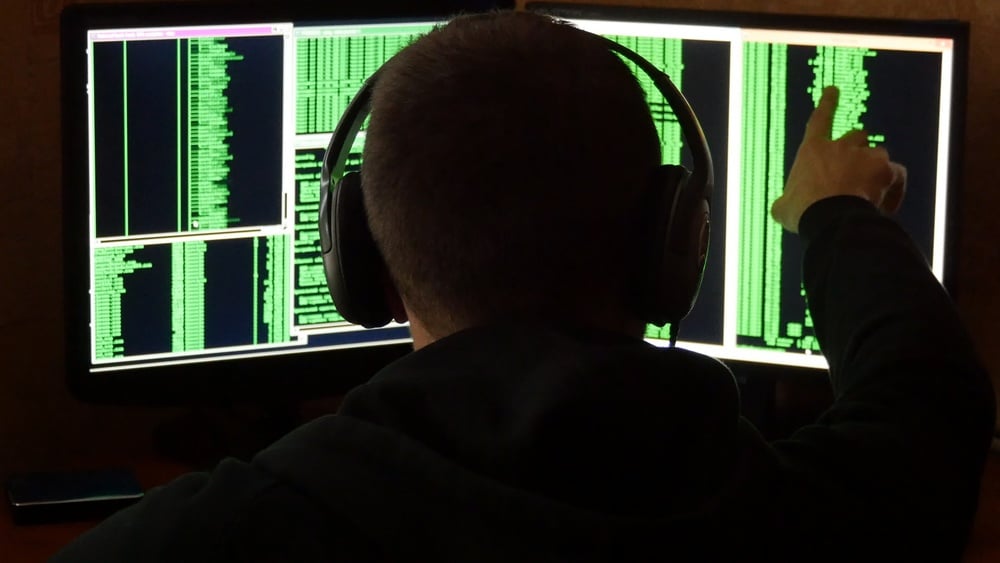
Recently, I heard someone express an opinion that “Quantitative analysis isn’t viable because we face intelligent adversaries.” This isn’t the first time I’ve heard this point of view, and I’m sure it won’t be the last. However, with this blog post I hope to provide a little better perspective on whether that point of view stands up well under scrutiny.
What is an “Intelligent adversary?”
Just so that we’re all on the same page, let’s identify a few key characteristics of “intelligent adversaries:”
- They are knowledgeable of the typical methods, practices, and technologies of their target.
- They are able to learn and adapt based on previous successes and failures — both their own and those of other attackers.
- They are able to create entirely new attack methods.
The underlying premise is that because we face intelligent adversaries in cyber risk scenarios, quantifying the risk associated with these scenarios is unreliable and a waste of time. Any estimates of risk will be inaccurate either because the bad actor(s) inherently change their methods/focus periodically, or because they anticipate our actions and adapt. Instead, the argument goes, we should stick to following well-established control best practices and/or limit our risk analyses to qualitative methods.
Broken logic and a failure to understand quantitative methods
There are several fairly obvious logical problems with the arguments against quantitative risk measurement and the suggested alternatives to quantitative analysis:
- How you measure risk has no bearing on whether an adversary is intelligent or not. Adversaries don’t become “unintelligent” when we use qualitative measurements.
- Slavishly aligning with “best practices” can arguably make an attacker’s jobs easier because they have a clear picture of the defender’s playbook.
- Qualitative measurements introduce significant limitations in an organization’s ability to prioritize effectively — e.g., out of a bucket of “high risk” issues, which is highest or lowest? Because of this inherent imprecision, most organizations struggle to identify and focus on the problems that matter most.
I suppose the grossly imprecise nature of High/Medium/Low qualitative risk ratings does appear to make them insensitive to changes in the landscape. What many people fail to recognize though, is that quantitative estimates expressed as ranges or distributions (which is how quantitative risk values should always be expressed) can be as imprecise as is necessary to faithfully reflect the dynamic nature of the risk landscape. For example: not at all sure about how frequently bad actors will attack your website? No problem — use a wider, flatter distribution to reflect that uncertainty. Oh, and by the way, if the landscape is so profoundly dynamic that you don’t think your uncertainty can be reflected quantitatively, then qualitative estimates aren’t going to be any better. In fact, qualitative ratings have additional limitations because it’s difficult to reflect potential variance across qualitative boundaries, and you generally never know whether the qualitative rating reflects best-case, worst-case, or something in-between.
The bottom line
For the life of me, I can’t figure out a logical argument for how the characteristics of intelligent adversaries are relevant to quantitative risk measurement but not to qualitative ratings, or that they somehow invalidate quantitative methods. The bad actors will still:
- Be knowledgeable of typical methods, practices, and technologies of their targets.
- Learn and adapt.
- Come up with new attack methods.
What is true, however, is that because intelligent adversaries add to the dynamic nature of the risk landscape, organizations need to update their analyses periodically. Of course, this is true for both qualitative and quantitative analyses. And despite common misperceptions, updating quantitative analyses is no more difficult than competently updating qualitative ones.
It seems clear to me that people who believe quantitative risk measurement isn’t viable because of “intelligent adversaries” either haven’t thought it through and/or they aren’t familiar with well-established quantitative methods like Monte Carlo, calibrated estimations, etc. Hopefully, this blog post clarifies things a bit.