Jack Jones: Automating Cyber Risk Quantification (Part 2 of 5)
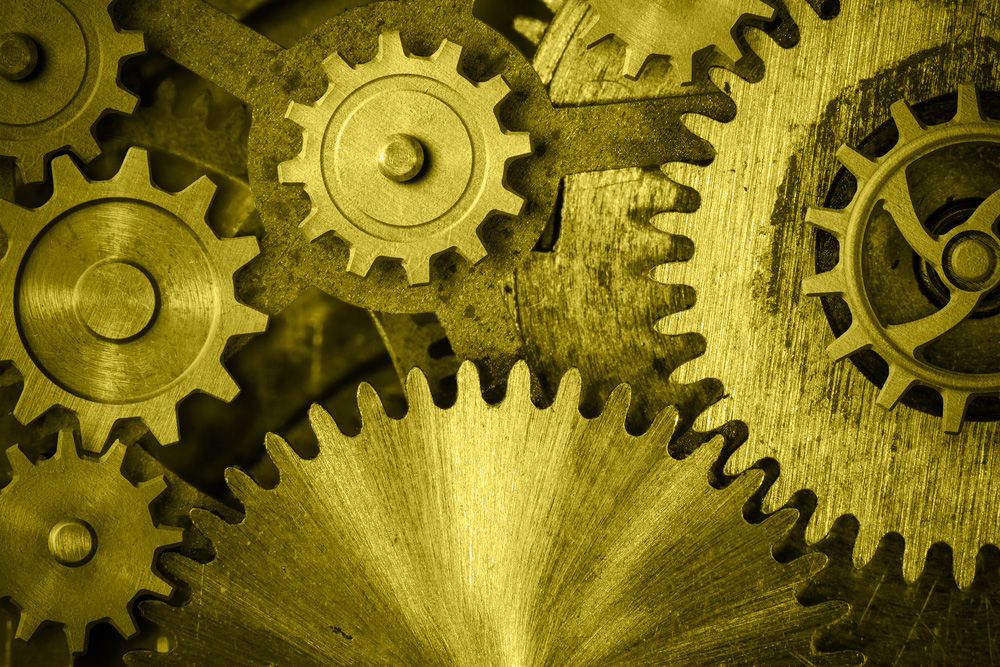
In Part 1 of this series, I discussed that the market for cyber risk quantification (particularly automated CRQ) is growing rapidly, but that automation, done poorly, can to more harm than good. In this post, I’ll begin to discuss what it takes to automate CRQ responsibly.
… are required for accurate CRQ (whether automated or not):
1. A clear scope of what’s being measured — e.g., the asset(s) at risk, the relevant threat(s), the type of event (outage, data compromise, fraud, etc.).
2. An analytic model (e.g., FAIR), which identifies the parameters needed to perform the analysis, and how data are used to generate a result.
3. Data, which can (ideally) be empirical data, or simply subject matter expert estimates.
On the surface, these don’t sound too intimidating, but all three need to be done well to get accurate results. And that isn’t as easy as one might imagine.
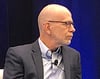
Scoping Quantitative Cyber Risk Analysis
It’s tempting to believe that scoping analyses would be simple. Just pick an asset (e.g., a web application), a threat (e.g., nation-state actors), and an event type (e.g., data compromise). Unfortunately, there’s often a lot of subtlety that must be accounted for to get accurate results. For example, does the scenario being analyzed involve code exploitation attacks, or attacks against authentication? The frequencies of these different attack methods may be very different, and some of the controls against them are significantly different. If we don’t make the distinction, the analysis results aren’t likely to be accurate.
Careful scoping also is crucial for risk aggregation, to avoid problems like double counting.
Automated CRQ solutions need to get scoping right, because if scoping is done poorly, it doesn’t matter how good the analytic model or data are — the results won’t be accurate. Fortunately, this can be addressed by either carefully pre-defining the scenarios, or by applying a scenario architecture that reduces the odds of error.
In the next post of this series, I’ll discuss the modeling component of CRQ automation.
Join the FAIR Institute, receive a free consultation with a FAIR Enablement Specialist