Manus AI Isn't the Risk, How You Use It Is: Navigating Autonomous AI with FAIR-AIR
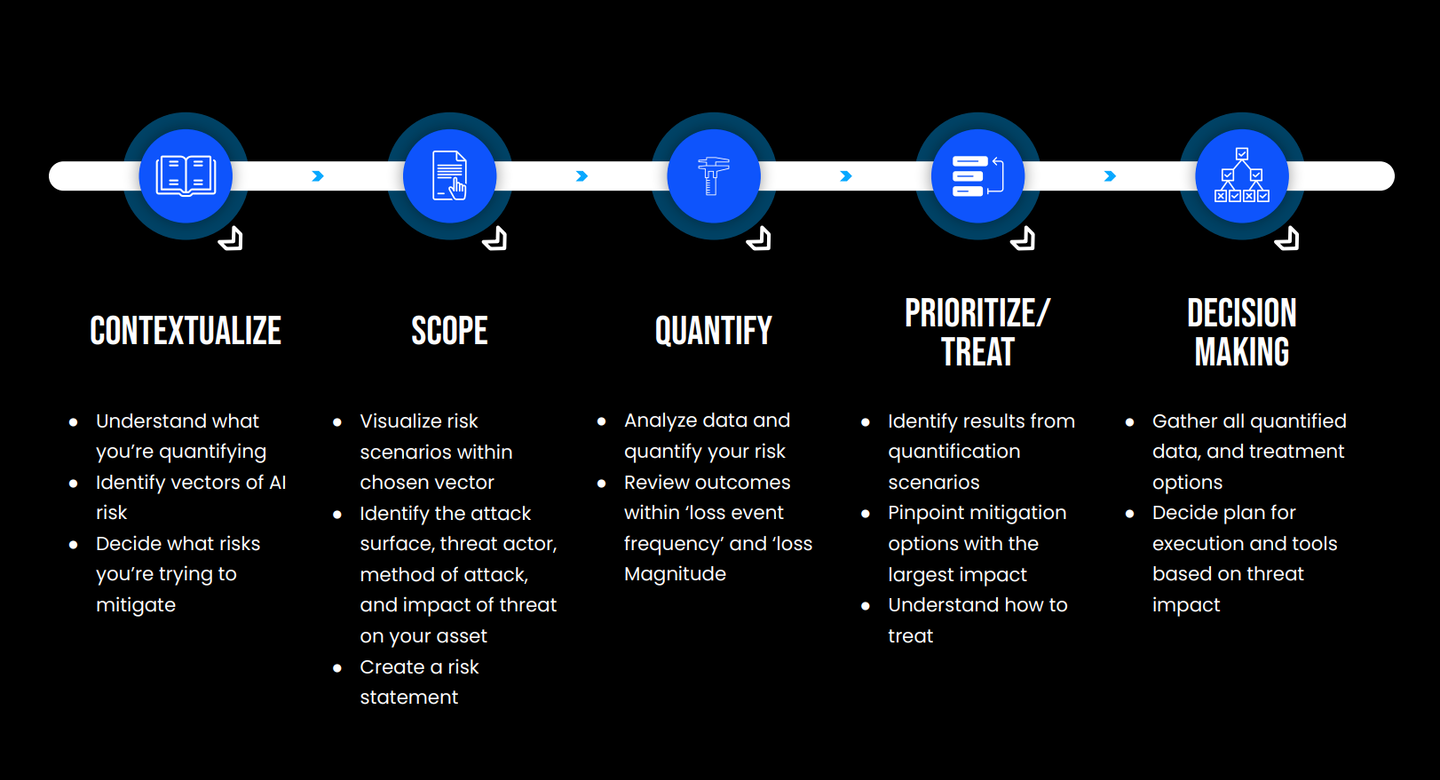
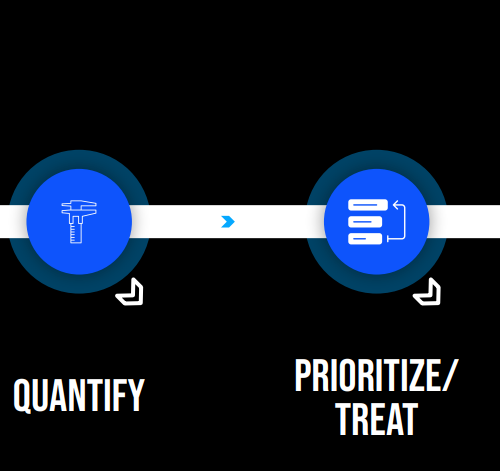
The buzz around Manus AI, the AI agent capable of autonomous task execution and logical reasoning, is palpable. It's the dawn of a new era, where AI can truly act independently.
But amidst the excitement, a familiar fear surfaces: "Is this safe?" I'm here to tell you that Manus AI, like any powerful tool, isn't inherently a risk. The risk lies in how we choose to wield it.
Let's cut through the hype and apply a structured approach: FAIR-AIR (Factor Analysis of Information Risk for AI). See the FAIR-AIR analysis steps in the image above. This framework helps us quantify AI-specific cybersecurity risks, moving beyond gut feelings to data-driven decision-making.
It All Starts with Business Context
Before deploying any AI, especially an autonomous agent like Manus AI, we must understand the business context. What tasks will it perform? How does this impact our existing cybersecurity risks?
High-Risk Use Case for Agentic AI: Autonomous Firewall Rule Modification
Business Context:
>>The organization wants to optimize network security by using Manus AI to dynamically adjust firewall rules based on real-time traffic patterns.
>>The goal is to enhance responsiveness to threats and reduce manual workload.
>>The risk is that an error in the AI's logic, or manipulation of the AI, could open the company up to attacks.
Possible Loss Scenarios for Manus:
>>Manus AI incorrectly identifies legitimate traffic as malicious and blocks it, causing a denial-of-service (DoS) event.
>>Manus AI incorrectly identifies malicious traffic as benign and opens firewall ports, allowing attackers to penetrate the network.
>>Attackers manipulate network traffic to trick Manus AI into opening unauthorized ports.
Loss Categories from the FAIR Materiality Assessment Model (FAIR-MAM):
Primary Loss:
>>Business Interruption: Network outages or slow performance disrupt business operations.
>>Network Security: Incident response, forensic investigation, and remediation efforts.
>>Replacement Costs: Replacing compromised network devices or software.
Secondary Loss:
>>Fines and Judgments: Regulatory fines for data breaches or non-compliance.
>>Reputation Damage: Loss of customer trust and brand value.
Control Mitigations:
>>Human in the Middle: Implement a mandatory review and approval process for all Manus AI-initiated firewall rule changes.
>>Anomaly Detection: Implement robust anomaly detection systems to identify suspicious network traffic patterns.
>>Rule Validation: Implement automated rule validation checks to ensure that Manus AI-generated rules don't create security vulnerabilities.
>>Rollback Capabilities: Ensure that firewall rule changes can be quickly and easily rolled back in case of errors.
>>Sandboxing: Test Manus AI's rule modifications in a sandboxed environment before deploying them to the production network.
>>Input Validation: Ensure that Manus AI is only able to use data that is trusted.
Depending on the controls implemented you may have higher risk reduction from one of these versus another. For instance, Human in the Middle would likely help mitigate the majority of these events from occurring but would also deteriorate the operational gains from this tool. So weighing these with a proper analysis will be important.
Low-Risk Use Case for AI Agents: Autonomous Vulnerability Scanning and Prioritization
Business Context:
The organization wants to improve its vulnerability management program by using Manus AI to automate vulnerability scanning and prioritization.
The goal is to identify and remediate vulnerabilities more quickly and efficiently.
The risk is that the AI could miss some vulnerabilities, or that the AI could provide false positives.
Possible Loss Scenarios:
>>Manus AI fails to identify a critical vulnerability, which is later exploited by attackers.
>>Manus AI generates a large number of false positives, wasting security analysts' time.
>>An attacker attempts to manipulate the data that Manus AI uses to scan for vulnerabilities.
Loss Categories (FAIR-MAM):
Primary Loss:
Productivity Loss: Security analysts spend time investigating false positives.
Network Security: Incident response and remediation efforts if a vulnerability is exploited.
Secondary Loss:
Fines and Judgments: Regulatory fines for data breaches caused by unpatched vulnerabilities.
Reputation Damage: Loss of customer trust if a data breach occurs.
Control Mitigations:
>>Human in the Middle: Require security analysts to validate Manus AI's vulnerability scan results before remediation.
>>Multiple Scan Engines: Use multiple vulnerability scanning engines to reduce the risk of missed vulnerabilities.
>>Regular Updates: Ensure that Manus AI's vulnerability database is regularly updated with the latest vulnerability information.
>>Weighted Risk Scoring: Implement a risk scoring system that considers multiple factors, such as exploitability and potential impact, to prioritize vulnerabilities.
>>Reporting and Tracking: Implement a system for tracking vulnerability remediation progress.
>>Data Validation: Ensure that Manus AI is only able to use data from trusted sources.
The FAIR-AIR Advantage in Autonomous AI Risk Analysis
Download the FAIR-AIR Approach Playbook
FAIR-AIR provides a structured way to:
>>Quantify Cybersecurity Risk: Instead of relying on subjective opinions, we can use data and probabilities to assess the potential impact of AI-driven cybersecurity actions.
>>Prioritize Cybersecurity Controls: By understanding the factors that contribute to cybersecurity risk, we can focus on implementing the most effective defensive measures.
>>Communicate Cybersecurity Risk: FAIR-AIR helps us communicate cybersecurity risk in business terms, facilitating informed decision-making.
Beyond the Hype on Manus AI
Manus AI, and other autonomous AI agents, represent a paradigm shift in cybersecurity. But we must approach them with a clear understanding of their risks and benefits. By leveraging FAIR-AIR, we can move beyond fear and embrace the potential of AI, responsibly.
1. The technology itself isn't the cybersecurity risk, the usage of the technology is
>>Don't fall into the trap of blanket bans or knee-jerk reactions to new AI tools. Focus on understanding how your teams intend to use them.
>>Instead of asking, "Is this AI safe?", ask, "What specific tasks will this AI perform, and how does it interact with our sensitive data and systems?"
>>Remember, AI is a tool. Like any tool, it can be used for good or ill. Your responsibility is to guide its usage towards the former.
2. Understand the cybersecurity business context
>>Don't deploy AI in a vacuum. Start by mapping out your organization's critical assets, data flows, and existing cybersecurity vulnerabilities.
>>Understand the specific business processes that AI will impact. What are the potential consequences of AI failure in these areas?
>>Align AI deployments with your overall cybersecurity strategy. AI should enhance your existing defenses, not create new blind spots.
3. Quantify the cybersecurity risk using FAIR-AI
>>Move beyond qualitative risk assessments. FAIR-AIR provides a structured, quantitative approach to understanding AI-related cybersecurity risks.
>>Use FAIR-AIR to model potential attack scenarios, estimate the probability of occurrence, and calculate the potential financial impact.
>>This data-driven approach will help you prioritize your security investments and communicate risk effectively to stakeholders.
>>When using AI, it is very important to document the assumptions, and to document the data that used. This will allow for easier FAIR analysis.
4. Implement robust cybersecurity controls.
>>Don't rely solely on the AI's built-in security features. Implement layered security controls, including access controls, data encryption, and intrusion detection.
>>Focus on controls that mitigate the specific risks identified in your FAIR-AIR analysis.
>>Establish clear policies and procedures for AI usage, including guidelines for data handling, model training, and incident response.
>>Ensure that there is a way to audit the actions of the AI.
5. Embrace responsible AI in cybersecurity.
>>Champion a culture of responsible AI development and deployment within your organization.
>>Prioritize transparency and explainability. Ensure that you can understand and audit the AI's decision-making processes.
>>Address the ethical implications of AI cybersecurity, particularly concerning data privacy and potential bias.
>>Stay informed about emerging AI threats and vulnerabilities. The AI landscape is evolving rapidly, and you must adapt your security strategy accordingly.
>>Prepare to respond to AI failures. Just like any other system, AI can fail. Have a plan in place to detect and respond to these failures.
Let's not fear the future, but rather shape it with informed, data-driven decisions.
Learn More:
AI in Cybersecurity: Governance and Risk Quantification in the Boardroom
Join the FAIR Institute! Start with a free Individual Membership.