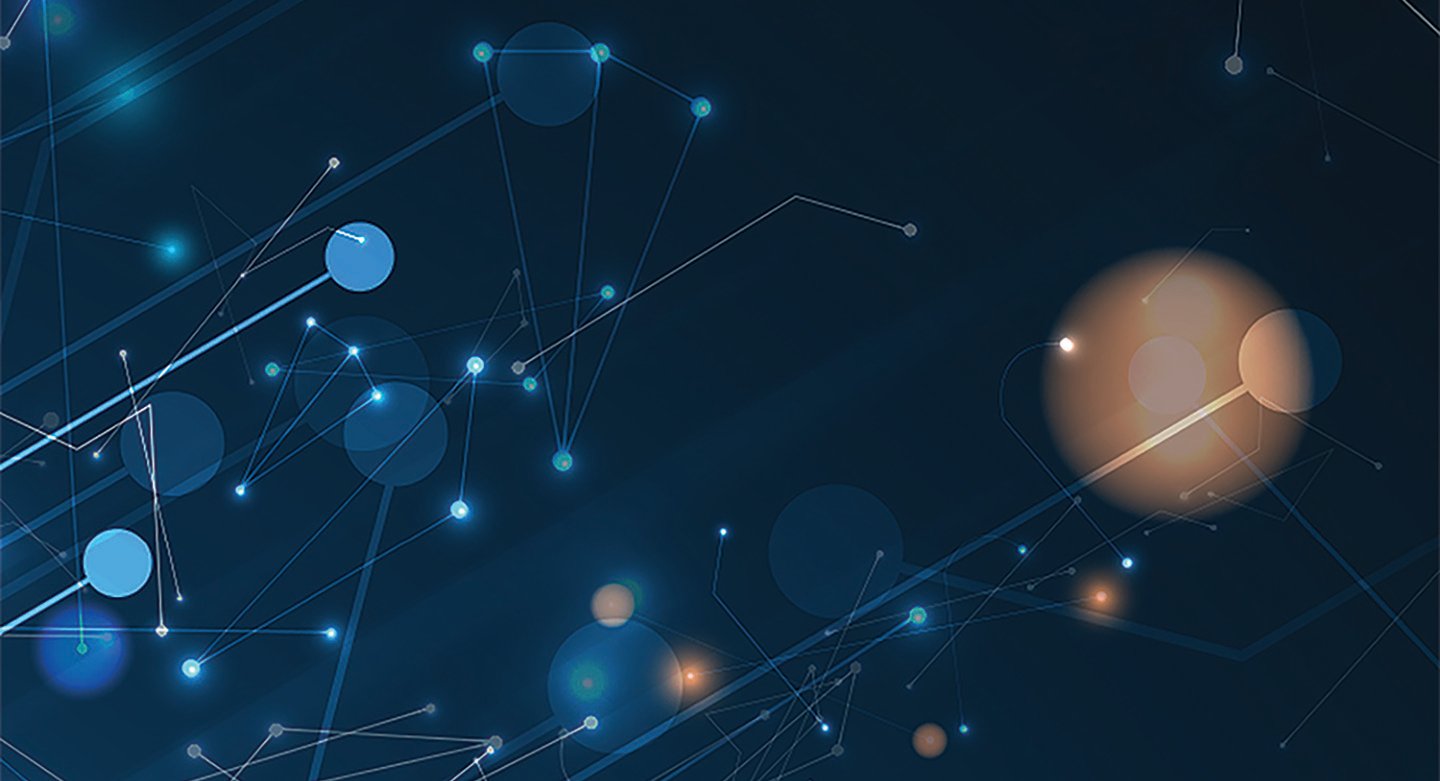
We get the question frequently these days: “What’s new and different about Generative AI risk?” We can analyze GenAI risk with our trusty FAIR model, but with new vectors, new threats and new loss categories. So, practically speaking, the short answer is: “It’s a completely new and different risk.”
GenAI is like electricity; it's all about how you use it. Here’s an analogy: Electrifying houses was great progress for humanity but also created a new risk of house fires. Firefighters had to learn to cut the power while smothering the flames. Similarly, the adjustment for GenAI is to understand how this new technology impacts your environment (like knowing to cut the power) and the new risks it produces.
AI RISK WEBINAR
Join author Jacqueline Lebo for a FAIR Institute webinar: "The Future of AI Risk Management: A Deep Dive with the FAIR Institute AI Workgroup" on Thursday, April 25 at 1 PM ET.
Education Break: What Is Generative AI?
The artificial intelligence field has been around for decades now. Generative AI is a field of Artificial Intelligence focused on creating entirely new content. This is accomplished by training a data processing model (AI model) on large amounts of data. The model will then identify patterns in the data and use that knowledge to generate content prompted by users or processes (though nobody knows exactly how that works).
Source: Wikimedia
FAIR Risk Analysis for GenAI
First, let's talk about scoping risk scenarios.
In FAIR, everything starts with a scenario. A well defined risk scenario at a minimum has an asset, threat, and effect. The easiest scenario to understand today is cyber criminals deploying ransomware in your environment leading to outages that impact your business and/or data loss. So, knowing our scenario is where we start with AI technology.
Key Point: Depending on what you are doing with AI and how much of the technology you are deploying yourself versus outsourcing will determine the types of scenarios you are most concerned about and what types of scenarios will be in scope for your solution. Unlike many emerging risks, AI risk comes with a variety of scenarios due to how versatile the technology is.
Next, how a GenAI risk scenario may look new and different from a pre-GenAI scenario:
Let’s take a “data poisoning” scenario. Pre-generative AI, a scenario might be: Copyrighted images get mistakenly added to a stock images library.
Probable losses:
- Response costs for internal teams to find the image and have it removed
- Compute costs to push the update to all Google instances
- Litigation expenses
Updated scenario for poisoning data: Copyrighted images are used in a data set to train an AI image-generating application. Depending on the validation controls you have in place and how quickly you can catch this will determine how much damage you have.
Probable losses:
- Compute and other costs to retrain the model, anywhere from $1M - $10M
- Response costs for internal teams to find the data set containing the image and have it removed and/or locate a version of the model before the copyrighted material is used.
- Litigation expenses.
Other new GenAI scenarios likely to become common:
- Prompt injection: A direct prompt injection occurs when a user injects text that is intended to alter the behavior of a large language model (LLM).
- Model theft: Steal a system’s knowledge through direct observation of their input and output, akin to reverse engineering.
- Data leakage: AI models leak data used to train the model and/or disclose information meant to be kept confidential.
- Hallucinations: Inadvertently generate incorrect, misleading or factually false outputs, or leak sensitive data.
- Insider incidents related to GenAI: For instance, insiders using unmanaged LLM models with sensitive data.
Download now:
Using a FAIR-Based Risk Approach to Expedite AI Adoption at Your Organization
Finally let’s review the controls for GenAI risk
For both the GenAI and non-GenAI scenarios, the techniques to review the data prior to creating the databases might look the same whether it be a third party managed software, third party data set, or manually created data set.
Non-GenAI example. After the data set is reviewed it would likely go into a test cycle that has less to do with the data in the database and more to do with the program to ensure its working correctly.
Additionally, developers would enforce data quality checks on batch and streaming datasets for data sanity checks, and automatically detect anomalies before they make it to the datasets. And capture and view data lineage to capture the lineage all the way to the original raw data sources.
Generative AI example. There are additional validation steps to ensure the trustworthiness of the generated responses where the AI software might produce copyrighted materials. Additional safety testing would also have the potential to catch the copyrighted material, however the main purpose of this testing would be safety requirements not necessarily data validation.
Benefits of FAIR Analysis for GenAI Risk Management
Generative AI presents exciting possibilities, but as we've seen, it also introduces unique risk factors compared to traditional AI. The potential consequences of data poisoning, for example, can be significantly amplified due to the model's ability to generate entirely new content.
The good news is that the FAIR framework provides a robust methodology for quantifying these risks and implementing appropriate controls. By integrating Generative AI risk assessments into your overall risk management strategy, you can:
- Identify and prioritize Generative AI-specific risks within your organization.
- Quantify potential losses associated with these risks, allowing for informed decision-making.
- Implement cost-effective controls to mitigate these risks and minimize their impact.
Remember, understanding how this new technology interacts with your environment is key. Proactive risk management is essential to harness the power of Generative AI while safeguarding your organization from potential pitfalls.
Next Steps
Attend the Webinar: "The Future of AI Risk Management: A Deep Dive with the FAIR Institute AI Workgroup" on Thursday, April 25 at 1 PM ET.
Read A FAIR Artificial Intelligence (AI) Cyber Risk Playbook