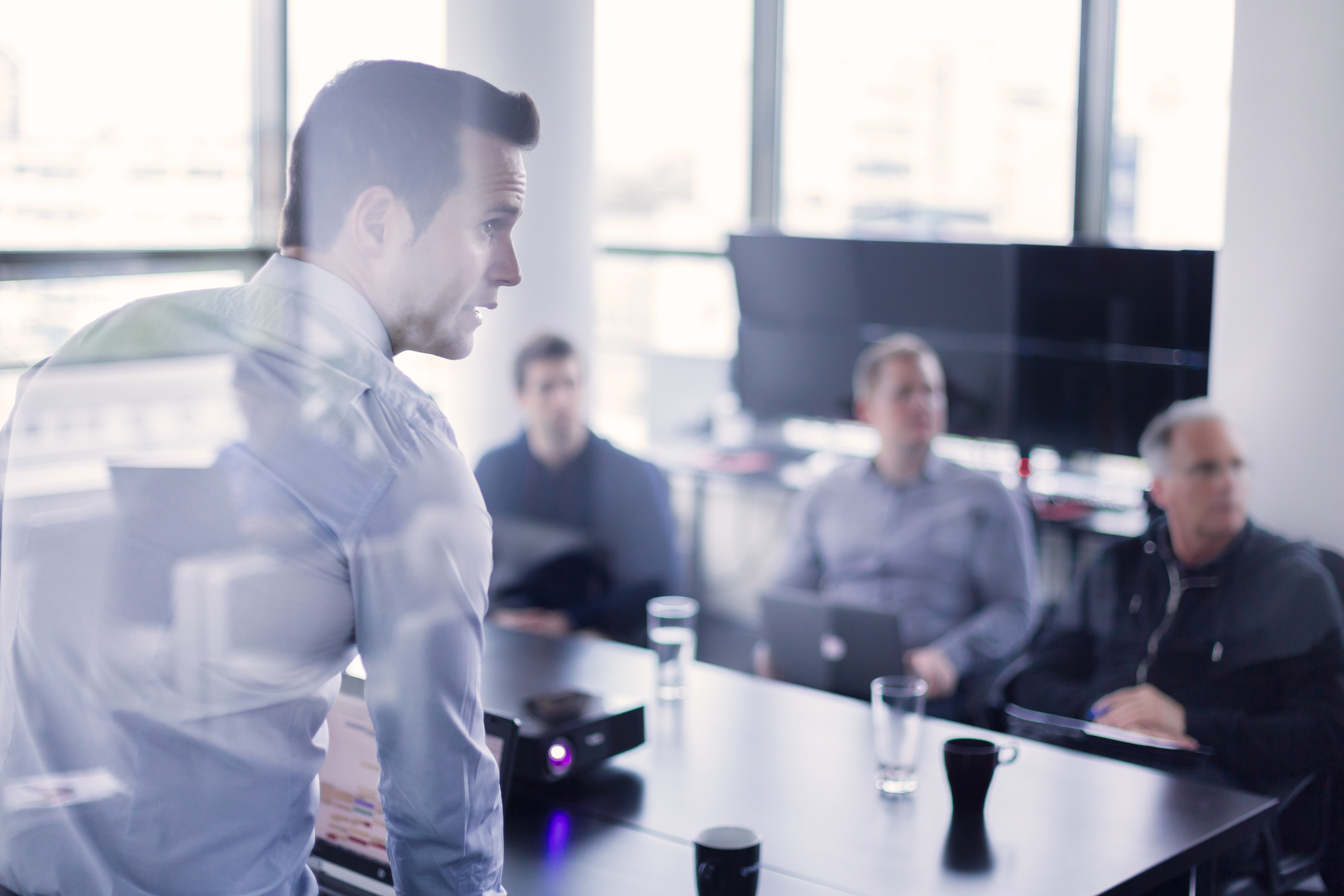
I recently spoke with a risk professional who had encountered challenges when presenting quantitative risk analysis results to business management. Specifically, his business colleagues struggled to “digest” the financial loss exposure values being presented to them — i.e., “What does it mean when you say there’s a one-in-50 chance of experiencing a $30 million dollar loss?” According to this risk professional, there seemed to be two primary concerns:
- From a frequency/likelihood perspective, since an event like this had never happened at the company, how did they come up with a 1-in-50 year probability?
- What does $30 million in loss mean? What about reputation damage?
It occurred to me that if, instead of quantitative values, someone had presented these business professionals with a risk rating of “high” for the very same analysis, and discussed the gaps in controls that existed, it's quite likely that nobody would have blinked an eye. Odds are, they’d all have pursed their lips and said something like, “Well, how long is it going to take, and how much will it cost to bring that down to Medium?” My experience has been that qualitative risk ratings are less likely to be questioned than quantitative analysis results, because they don't expect you to have answers to their questions.
This is unfortunate for a number of reasons, including:
- Qualitative ratings rarely have any rigor behind them and are thus far more likely to fall apart if looked at closely.
- Everyone who hears terms like "High risk" or "Significant reputation damage" or "Highly likely" are going to interpret them differently. Unless, of course, those terms are defined quantitatively (e.g., "Highly likely equals greater than 90% probability of occurring in the next 12 months.")
But be that as it may, let’s discuss the two concerns.
Frequency/likelihood
There are a lot of people who believe that you have to have actuarial data in order to accurately estimate the occurrence of some future event. Nice idea, but the simple fact is that such data (almost by definition) doesn’t exist for low frequency events. Furthermore, two additional considerations make it unrealistic to rely on empirical data for many of the scenarios we face:
- The cyber risk landscape changes rapidly, which can limit the useful lifetime of empirical data.
- The cyber industry hasn’t historically shared loss eventfrequency data in any meaningful way. Certainly not in volumes that are statistically meaningful, and not in ways that can easily be applied to a specific organization.
Unfortunately, the fact that we don’t have good actuarial data for low frequency events doesn’t mean that we get to ignore the frequency part of risk. It’s there whether we like it or not, and it’s crucial to include if we hope to make well-informed risk decisions. For example, without considering frequency, a $10M event that occurs once every five years would be the same as one that occurs once every one hundred years.
Some people will, as a proxy for frequency, simply talk about controls — with the belief that more/stronger controls equals lower frequency. And while there is often a correlation between loss event frequency and controls, that approach is a bit like deciding not to buy life insurance because you always buckle your seatbelt.
Explaining loss event frequency estimates to skeptics is an important skill to have if you want to be effective in this field. I’ve found it helpful to briefly include three things in my explanation:
- The rationale (data and assumptions) that helped to inform the estimate.
- The fact that estimates are expressed as ranges and/or distributions, which allows us to reflect the quality of data we’re operating from and our level of certainty in the estimates.
- The quality of estimates are improved by using Calibration methods, and that these methods have been proven through rigorous studies.
More often than not, this will quell concerns. That said, sometimes you may still encounter a refusal to engage in meaningful dialog/debate. In those cases, I’ll often wonder whether this same person would apply a qualitative likelihood rating without admitting that the data underlying that rating is no better (and probably worse) than what would inform a quantitative estimate.” Perhaps it’s the inherent ambiguity and subjectiveness of qualitative values that appeals to them.
Loss magnitude
It isn’t unusual to run into business colleagues (and even some risk professionals) who are so used to qualitative descriptions of impact that they struggle to think in quantitative terms (e.g., “If this ‘risk’ materializes, there could be significant reputation damage and severe customer impact.”). This should be a very easy hurdle to overcome if you’ve done your homework during the analysis. Whatever the quantitative loss magnitude estimate is, it should have been arrived at by evaluating each of the forms of loss within FAIR, which includes reputation damage, response costs, etc. All of these should be easily understood by anyone who is a legitimate business professional.
It is also important that the loss magnitude estimates in an analysis come from business subject matter experts. Need an estimate on fines and judgments? Speak with colleagues in legal and/or compliance. Want to understand the potential for lost market share? Discuss it with colleagues from sales and marketing. This not only helps to ensure that the numbers are accurate, but it provides credibility in the face of skepticism.
Be prepared
I've also encountered organizations where the CISO (or other risk executive) is hesitant to put quantitative analysis results in front of senior business executives because the CISO wasn't closely involved in the analysis or is only marginally familiar with FAIR. Because of this, they aren't confident that they can field the questions that might be asked. That's actually a good call. The last thing you want to do is stumble through an explanation of the results and how they were arrived at. Fortunately, the antidote for this is (or should be) simple. Either include someone from the team to the presentation who is able to explain things clearly and simply, have the CISO come up to speed, and/or translate the quantitative results into a qualitative scale.
At the end of the day
Overcoming the inertia that stems from the qualitative and superficial thinking that surrounds cyber and operational risk today is one of the biggest frustrations you may face. My suggestion is to take a deep breath and keep forging on. The trend in the industry toward quantitative methods is clear, and we’re making great progress. Eventually, smart horses will learn to drink.