Assessing and Addressing AI Risks: A Triage Approach with the OWASP Top 10 for LLMs
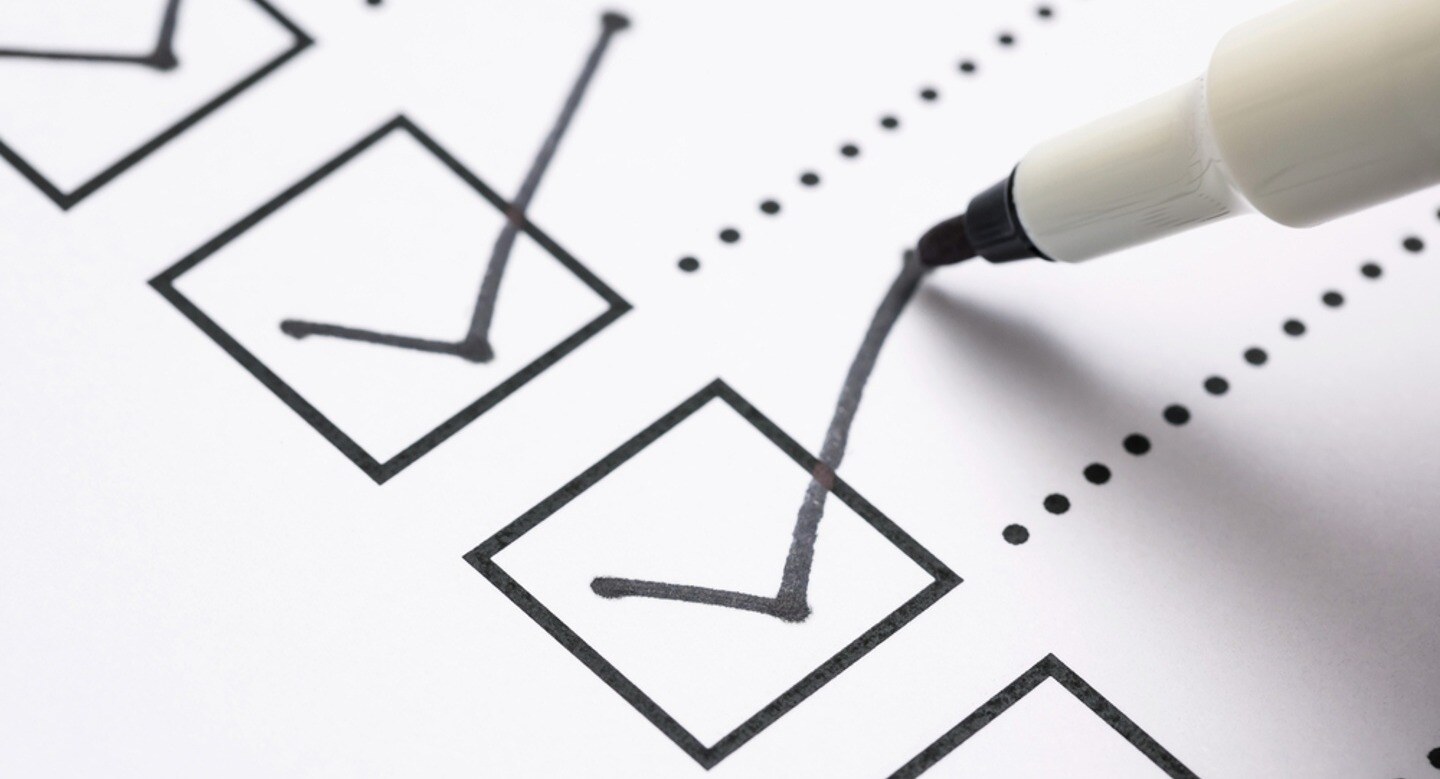
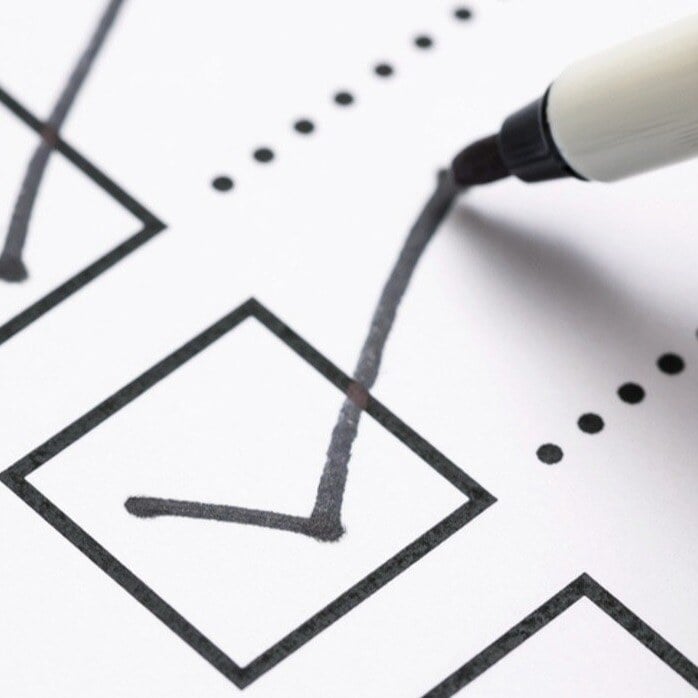
In an era where artificial intelligence (AI) plays an increasingly vital role in various industries, understanding and managing AI risks is paramount. Generative AI, in particular, introduces unique challenges and opportunities that necessitate thorough risk management practices.
This blog post aims to demystify AI risk, demonstrate how to use the OWASP LLM Applications Cybersecurity and Governance Checklist, and invite the risk community to share their insights on determining the materiality of AI risks.
The FAIR Institute AI Workgroup is:
Jacqueline Lebo, Risk Advisory Manager (Safe Security)
Arun Pamulapati, Sr. Staff Security Field Engineer (Databricks)
Brandon Sloane, Security Risk Management Lead
AI Risk at the 2024 FAIR Conference
Arun and Jacqueline will lead a workshop “Mastering AI Governance and Risk Management” at the 2024 FAIR Conference, 1-5 PM, Monday, September 30, in Washington, DC. FAIRCON24 will feature many other discussion sessions on AI-related risk. Register now for the FAIR Conference.
What Is AI Risk?
AI risk encompasses potential threats and vulnerabilities that arise from the development, deployment, and operation of AI systems. These risk themes can range from data privacy concerns and model bias to operational failures and adversarial attacks.
The unique nature of AI, especially generative models, amplifies these risk themes due to their ability to create new content, learn from vast datasets, and adapt over time. Whereas, if you look at how these different themes intertwine, you will start to be able to understand how likely a data privacy event is based on applicable threats, vulnerabilities and how losses materialize based on the business case.
The OWASP LLM AI Cybersecurity & Governance Checklist
The OWASP LLM AI Cybersecurity & Governance Checklist is a comprehensive tool designed to help organizations identify and mitigate AI risk. The checklist covers a wide array of considerations, including data management, model security, ethical implications, and compliance requirements.
Several key areas from the checklist:
- Data Governance: Ensures the integrity, privacy, and security of data used in AI models.
- Model Governance: Addresses the development, deployment, and monitoring of AI models to prevent bias, ensure fairness, and maintain transparency.
- Operational Security: Protects AI systems from adversarial attacks and operational disruptions.
- Compliance and Legal: Ensures adherence to relevant regulations and standards.
- Ethical Considerations: Evaluates the ethical implications of AI applications and their impact on society.
Diving Deeper into the Checklist
The checklist is divided into thirteen focus areas for risk analysis. Here are the most critical points from each area that your risk and security team should consider:
Adversarial Risk
- Competitor and Attacker Analysis: Evaluate how competitors are using AI and assess potential threats from adversaries.
- GenAI Attack Mitigation: Update incident response plans to address AI-enhanced attacks and AI/ML incidents.
- Transparency and Accountability: Document and communicate incident management activities to stakeholders.
Threat Modeling
- Comprehensive Assessment: Threat model the entire AI system, breaking it down into components and categorizing AI deployments.
- Threat Identification: Identify specific threats related to AI models, such as adversarial examples, poisoning attacks, and model stealing attacks.
- Continuous Monitoring: Stay updated on the latest research and methodologies to address emerging threats.
AI Asset Inventory
- Comprehensive Catalog: Maintain a detailed inventory of all AI assets, including data, models, and infrastructure.
- Risk Assessment: Prioritize assets based on their criticality and potential risk exposure.
AI Security and Privacy Training
- Awareness and Education: Provide training to staff on AI-specific risks, security best practices, and privacy regulations.
Establish Business Cases
- Justify Investments: Develop clear business cases for AI security initiatives, demonstrating their value and return on investment.
Governance
- Clear Roles and Responsibilities: Define roles and responsibilities for AI governance and risk management.
- Decision-Making Framework: Establish a framework for making informed decisions about AI risks and mitigation strategies.
Legal and Regulatory
- Compliance Assessment: Ensure compliance with relevant regulations and standards, such as GDPR, CCPA, and industry-specific requirements.
Using or Implementing Large Language Model (LLM) Solutions
- Risk Assessment: Evaluate the specific risks associated with using or implementing large language model solutions.
- Mitigation Strategies: Develop strategies to address identified risks, such as data privacy, bias, and security.
Testing, Evaluation, Verification, and Validation (TEVV)
- Rigorous Testing: Conduct thorough testing of AI models to identify and address vulnerabilities.
Model Cards and Risk Cards
- Transparency: Develop model cards and risk cards to document the key characteristics, limitations, and risks of AI models.
RAG: Large Language Model Optimization
- Continuous Improvement: Utilize RAG frameworks to optimize large language models and address identified risks.
AI Red Teaming
- Simulated Attacks: Conduct red teaming exercises to test the resilience of AI systems against adversarial attacks.
Using the FAIR-AIR Approach Playbook to Define Your AI Risk Scenarios
The FAIR Institute’s AI Workgroup created the FAIR-AIR Approach Playbook to give risk managers and security teams a high-level way to get their arms around the Gen AI risk problem space. The Playbook takes a FAIR-influenced approach that complements the OWASP Checklist.
Principally, you can use the “Contextualize” steps in the Playbook to define your key risk scenarios and clarify the quantitative, frequency-and-magnitude inputs, then move on to prioritize and treat, leveraging the more granular parameters of the OWASP Checklist.
Download the FAIR-AIR Approach Playbook.
Using the OWASP Checklist to Identify Key AI Risks to Quantify
To quickly determine which AI risks to quantify, follow these steps:
- Identify High-Impact Areas: Focus on areas of the checklist that align with your organization's critical operations and data sensitivity. For example, if your AI application handles sensitive customer data, prioritize Data Governance, Compliance, and Operational Security.
- Assess Model Complexity: Consider the complexity and scope of your AI models to identify potential risks.Generative models, due to their sophisticated nature, may require a more in-depth risk assessment focusing on Model Governance and Operational Security.
- Evaluate Regulatory Environment: Identify relevant regulations and legal standards to prioritize compliance-related risks.
- Map Checklist Gaps to Critical Risks: Once you've identified high-impact areas, map the corresponding checklist gaps to critical risks. For example, if you have a gap in red teaming, assess how that might increase your LLM's vulnerability to threat actors.
- Collect Data to Quantify Risks: Gather relevant data to quantify the identified risks. This might include:
>>Historical data: Analyze past incidents, breaches, or near-misses to understand the potential impact of specific risks.
>>Industry benchmarks: Compare your organization's risk profile to industry benchmarks to identify areas of concern.
>>Expert assessments: Consult with experts to obtain qualitative insights into the potential consequences of certain risks.
>>Quantitative metrics: Use metrics like mean time to repair (MTTR), mean time between failures (MTBF),and financial loss estimates to quantify risks.
- Prioritize Remediation Efforts: Use the quantified risk data to prioritize remediation efforts, focusing on the most critical gaps that pose the greatest threat to your organization.
By following these steps, you can effectively use the OWASP AI Security and Governance Checklist to identify and quantify key AI risks, enabling you to make informed decisions about resource allocation and risk mitigation strategies.
Engaging the Risk Community
Determining the materiality of AI risks is a nuanced process that benefits from diverse perspectives. We invite the risk community to share their methodologies and criteria for assessing the materiality of AI risks. How do you determine which risks are significant enough to quantify? What frameworks or tools do you use to guide your assessments? Your insights will contribute to a broader understanding of AI risk management and help organizations navigate this complex landscape more effectively.
Join the conversation and share your thoughts on AI risk materiality in the comments below or by completing the linked survey. The AI Workgroup will publish all research findings for public consumption after the close of the survey, August 30, 2024.
Conclusion
As AI continues to evolve, so too must our approaches to risk management. By leveraging tools like the OWASP AI Security and Governance Checklist and engaging with the risk community, we can develop more robust strategies to quantify and mitigate AI risks. Together, we can ensure that the transformative potential of AI is realized safely and ethically.