Calibrated Estimation for FAIR™ Cyber Risk Quantitative Analysis - Explained in 3 to 4 Minutes
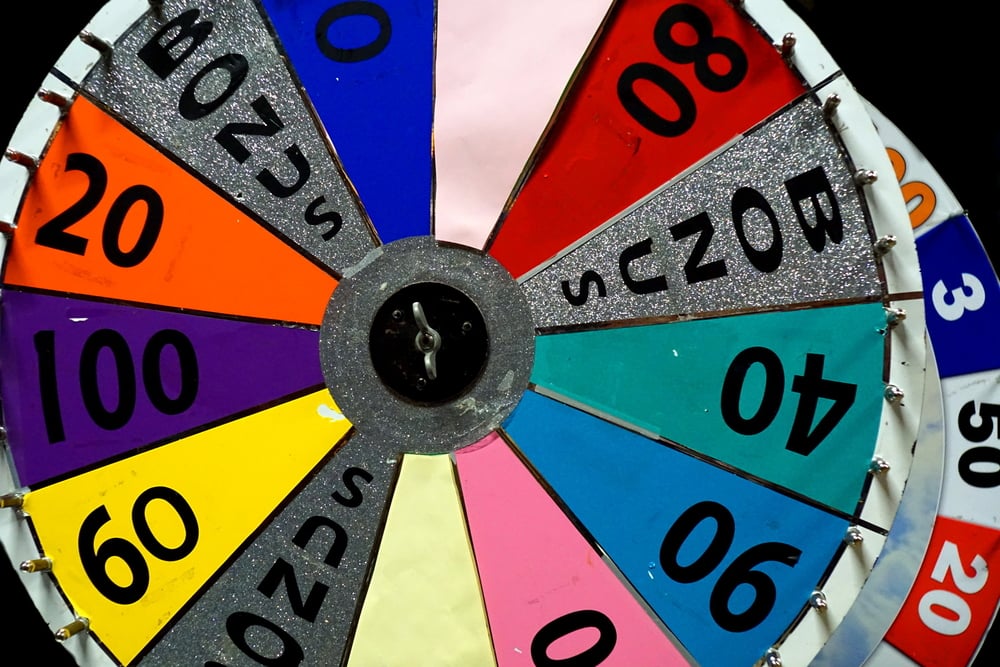
Cyber risk quantification has often been seen as difficult or impossible due to the perceived lack of data on the subject. Many organizations do not have sophisticated logging systems which allow them perfect hindsight into past cyber events.
So how can we begin to measure our cyber risk exposure in light of such uncertainty?
But wait..What does the word “measure” even mean for cyber risk?
Often people assume that to measure means to determine a singular precise value. In actuality, measurement is simply about reducing uncertainty, not achieving precision.
Due to the fact that when conducting quantitative risk analysis, we are always looking forward, there will always be some degree of uncertainty in our estimations.
“Although this may seem a paradox, all exact science is dominated by the idea of approximation. When a man tells you that he knows the exact truth about anything, you are safe in inferring that he is an inexact man. Every careful measurement in science is always given with the probable error (…) every observer admits that he is likely wrong, and knows about how much wrong he is likely to be.” --Bertrand Russell, mathematician and philosopher |
With that thought in mind, in FAIR™ quantitative risk analysis, we estimate using ranges that are
- Accurate and
- Usefully precise
The way we achieve accuracy with a useful degree of precision is using a technique called Calibrated Estimation. From the Open FAIR™ standard: “Calibration is a method for gauging and improving an individual’s ability to make good estimates. Because measuring risk involves making good estimates, calibration is critical for risk analysts to understand.”
The 4 Steps of Calibrated Estimation
Calibrated estimation is a four-step process that enables you to reduce cognitive biases and provide accurate estimations, even in instances of little to no data.
-
Start with the absurd
-
Eliminate highly unlikely values
-
Reference what you know to narrow the range
-
Use the equivalent bet method to gauge your confidence
Step 1, start with the absurd, is intentionally designed to help the analyst get out of the “I have no idea!” mindset, while also discouraging anchoring – a common bias in estimation of over-weighting the first bit of information received.
Steps 2-3 are geared toward using logical reasoning and external references to narrow the original range.
Finally, step 4 aids in the analyst in achieving that “useful” degree of precision.
What is the equivalent bet method?
Imagine you are at a carnival. There is a giant spinning Wheel of Fortune with nine green sections and one red. If you spin the wheel and land on green, you win $1000. If you land on red, you lose. Each time you get to step 4, you are given the option to bet on their range or spin the metaphorical carnival wheel. If you immediately choose the wheel, that means you are less than 90% confident in your range as you believe you have better odds of winning playing the game. In order to increase your confidence, you need to widen your range to increase your odds of accuracy.
If you immediately choose your range, it means you have greater than 90% confidence in your range. This means that while your range is likely accurate, it may be too wide to be usefully precise and can be narrowed further.
The game continues until you cannot immediately choose between either option – at which point you are 90% confident.
Calibrated Estimation in Action
Let’s say you are estimating how much risk your organization is exposed to as a result of external malicious actors compromising customer Personally Identifiable Information (PII) contained in your crown jewel database. Your first step is determining how often this event may occur. As it has not happened historically, you are estimating the number of attempts (Threat Event Frequency) and the likelihood those attempts will be successful (Vulnerability).
To start with an absurd range, you estimate that attempts are occurring between once in every five years and 100 times per year. Knowing the database contains highly sensitive data and that due to your industry, attackers would be aware of this as well, you determine it is more reasonable that at least once every two years this type of attempt would occur. Given that there are no known database breaches of this type historically, you feel confident in reducing your maximum to 1-2 per month, or 24 times per year.
Then, after thinking through where the database sits (internal, in a segmented network), you reason that in order to attempt to compromise this database, the attacker would first have to access the network and then pivot to this database. You’re aware of network intrusions happening in the past, at least 1-2 per year, and while your monitoring systems aren’t perfect, you are confident that these successful network intrusions are not happening more than once or twice a quarter, bringing your maximum down to 8 times per year. You play the equivalent bet game until you get to a range you are 90% confident in – .5 – 6 times per year.
For more on calibrated estimation, check out the books How to Measure Anything and/or How to Measure Anything in Cybersecurity Risk by Douglas Hubbard. And watch the FAIRCON19 video of Hubbard’s presentation on Overcoming the Myths of Cyber Risk Measurement. Also, see this blog post by FAIR creator Jack Jones: No Data? No Problem.
Looking for training in calibrated estimation and other techniques of quantitative cyber risk analysis? Contact the FAIR Institute’s FAIR Enablement Specialists Team.