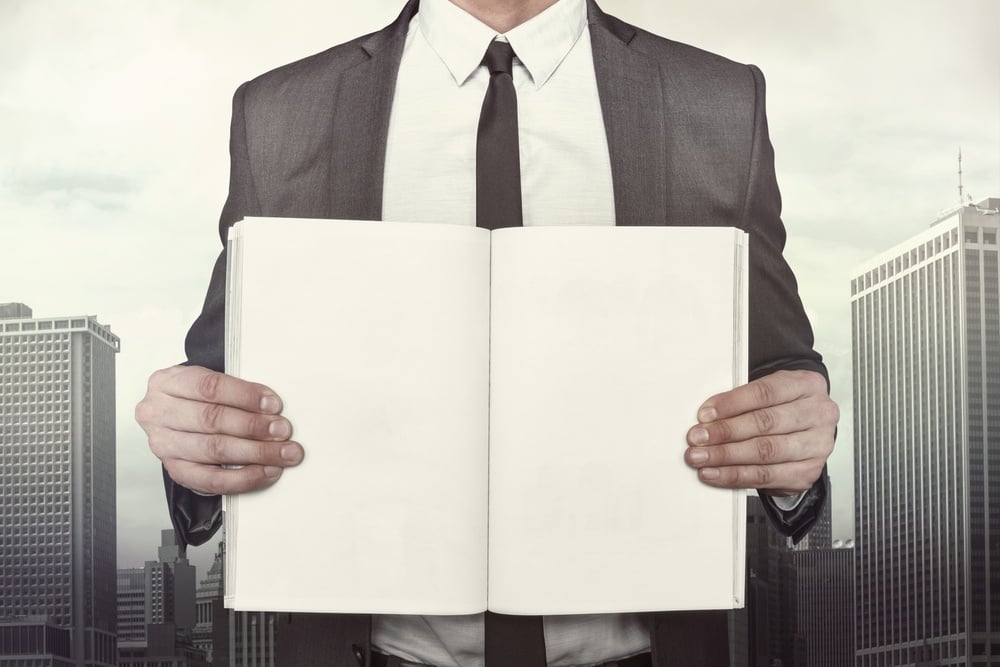
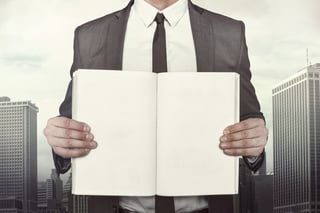
Dealing with lack of historical data in risk assessments
It seems a lot of people believe that in order to make an accurate estimate of some future event (e.g., loss event likelihood, or the consequences of an event), you need boatloads of empirical data. Sure, data is nice to have when it’s available, but what if data is sparse or nonexistent? You still have to somehow deal with whatever measurement question you face. As a result, you can:
A) Use a qualitative measurement like “Low” and essentially sweep under the rug your lack of data and the associated uncertainty in your results, or
B) Completely exclude the measurement in question from your decision-making (e.g., simply focus on impact, if likelihood is in question, or vice-versa), or
C) You can use well-established methods like calibrated estimation, expressing estimates using ranges or distributions, and Monte Carlo functions.
Option “A” is easiest, and it’s what people default to when they don’t know any better or if they have to give an off-the-cuff answer “right this instant”. Option “B”, in my opinion, is severely misguided in most cases. In fact, I’ve done experiments using agent-based modeling that suggest option “B” is roughly equivalent to flipping a coin in terms of actually managing an organization's loss experience. Option “C” requires a little more work (usually much less than you think) but it allows you to improve the odds of an accurate answer (options “A” and “B” are fraught with the potential for error). Option “C” also allows you to faithfully express the quality of data you’re operating from, and thus your uncertainty, by expressing your estimates using wider, flatter distributions as appropriate.
Measuring anything in cyber risk
I encourage people who struggle with this question of “no data” to pick up Douglas Hubbard’s books, How to Measure Anything and/or (more recently) How to Measure Anything in Cybersecurity Risk. A few of my favorite quotes from Douglas include:
- You have more data than you think you do
- You need less data than you think you do
- There is literally nothing we will ever need to measure (estimate) where our only bounds are negative infinity to positive infinity
This last one is especially relevant because it speaks directly to the “I have no idea” reaction that a lack of data often elicits. I’ve been faced with that reaction dozens of times over the years as I’ve trained people in risk analysis and estimation, and 100% of the time they DO have an idea. Often a pretty good idea. They just don’t know how to approach it.
Other good sources of information would be any basic book on Bayesian reasoning and analysis, as well as the book I co-authored, Measuring and Managing Information Risk: A FAIR Approach.
A simple example I’ll sometimes use to demonstrate my point is to ask someone in an audience to estimate my wife’s height. Here’s how that conversation commonly goes:
Them: “Huh? I have no idea! I’ve never met or even seen her.”
Me: “Well, is she less than an inch tall, or more than ten feet tall?”
Them: “Of course not. Nobody is.”
Me: “So you do have some idea. How about less than three feet tall or more than seven feet tall?”
Them: “Probably not, but it’s not impossible.”
The conversation continues with me helping them to continue narrowing their range using various references and logical considerations. And every time they narrow their range I ask them to choose between their range being accurate (i.e., containing my wife’s actual height) versus spinning a carnival wheel where they have a 90% chance of winning $1,000. BTW — Douglas does a great job of describing this “equivalent bet” approach in his books.
The bottom line is that they can always arrive at a range they have 90% confidence in — without ever meeting or seeing my wife — and the same process can be used to estimate any value where you believe you have no data. Some of the keys to this calibrated estimation process include:
- Start with an absurd estimate (e.g., less than an inch or greater than ten feet tall). It breaks the ice and gets people out of the “I have no idea” mindset. It also helps to compensate for anchoring, which is a cognitive bias people often suffer from when estimating.
- Use references and logical reasoning to begin narrowing the range.
- Use the equivalent bet method to continually test your confidence as you narrow the range.
- Challenge your reasoning along the way, and consciously look for reasons your range might be wrong.
- Remember that accuracy — not precision — is king. Many people gravitate toward precision, but that’s a great way to end up with an inaccurate answer.
Although this approach doesn’t guarantee accuracy, studies have shown that it significantly improves estimate quality. Furthermore, it inherently allows you to express your confidence — wider ranges represent lower confidence. This is a grossly underappreciated facet of risk measurement that qualitative measurements almost never convey.
At the end of the day, in this profession we very often have to analyze risk-related scenarios where we have little data. This is simply a fact of life that doesn’t change by falling back on options “A” or “B”. By leveraging well-established quantitative methods, we can improve the accuracy of our analyses and also convey confidence levels.
BTW – some of you may be asking yourself the question: "What about unknown unknowns?" As it turns out, I've already written a blog post about that (read it here).
Stay tuned for a discussion of “historical data”…