Jack Jones: Automating Cyber Risk Quantification (Part 4 of 5)
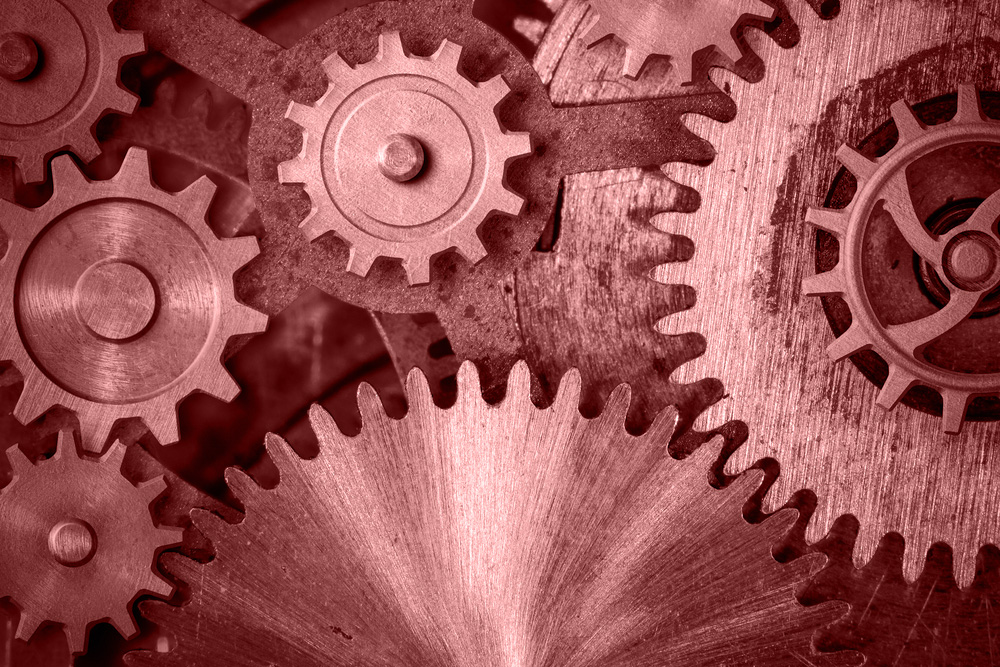
I covered a lot of ground in the previous posts, and rather than summarize them here I’ll assume you’ve read those posts already. So, let’s dive into the last analytic dimension…
Data
Unlike the scope and model dimensions, where deficiencies are commonly fatal in terms of their effect on analysis accuracy, there’s more room for imperfection in data. That’s in large part because there are well-established methods for dealing with sparse data — things like Monte Carlo and other stochastic functions that exist specifically to deal with data-related uncertainty, as well as calibration methods that dramatically improve the quality of subject matter expert estimates. That’s the good news.
Jack Jones is the creator of FAIR™ (Factor Analysis of Information Risk), the international standard for quantification of cyber, technology and operational risk, as well as the FAIR Controls Analytics Model (FAIR-CAM™) that quantifies how controls affect the frequency and magnitude of risk.
But wait, there’s even more good news. We’re finally beginning to see significantly better loss magnitude data (unfortunately, that’s because there have been so many breaches to gather data from), and threat related data also continues to improve. Which just leaves controls related data, and we have a ton of that, right? Well, yes and no. Yes, the cybersecurity industry does generate a lot of controls related data (more of that, in fact, than loss or threat data). But no, in many cases the data are simply not usable for CRQ.
But didn’t I just say that there’s more room for imperfection in data? Yes, but imperfection in terms of volume (sparseness) is very different from imperfection in terms of accuracy. If the sources of our data are fundamentally flawed, and particularly if there isn’t a pragmatic way of separating the good from the bad, then using those data in CRQ will inevitably lead to unreliable results. Let’s look at two primary examples of this…
CVSS Scores
The simple fact is that the CVSS scoring model involves a lot of math on ordinal values. Functionally, that’s the same thing as doing math on a color scale. In order to be quantitative, there has to be a unit of measurement — a quantity of something — like frequency, percentage, monetary values, time, etc. If there is no unit of measurement, a numeric value isn’t quantitative. In a numeric ordinal scale (e.g, 1-5 or 1-10), the numbers are simply labels for buckets/categories, just as colors would be. Yes, I know “almost everyone in cybersecurity does math on ordinal scales”, but there was a time when almost everyone in medicine practiced blood-letting. Volume of use doesn’t always correlate with efficacy.
Math on ordinal values is only one of several problems with CVSS scores, but it is significant enough by itself to invalidate them as reliable data. From a CRQ perspective, these problems mean that CVSS scores can’t reliably be translated into quantitative values. And by the way, this isn’t a “precision problem” — it’s an accuracy problem. We could live with it if it just affected measurement precision.
Learn FAIR risk analysis with training approved by FAIR Institute. Courses offered live and online. Become FAIR Trained and Certified and change how you, your team, and your organization view and act on risk.
NIST CSF Scores
NIST CSF has gained a lot of traction as a means of gauging and communicating about cybersecurity programs at a high level, and I’m not here to debate its efficacy for those purposes. However, it has several characteristics that make it inappropriate as a source of control-related data for CRQ. For the sake of brevity, I’ll just discuss two of them:
- There is no standard scoring model for NIST CSF. The 1 - 4 ordinal Tier scale that NIST defines within the CSF is intended to characterize a cybersecurity program overall, and not the individual subcategories. Consequently, organizations are free to define whatever scoring scale they please for the subcategories. This means that a “2” for one organization may not equate to a “2” for another organization. For that matter, some organizations may use a five-level scale, while others use four levels. This makes it impossible to reliably translate scores for use within CRQ, at least in an automated fashion.
- Many of the CSF subcategories are too broadly written to be reliably scored for CRQ purposes. For example, PR.AC-1 is defined by CSF as: “Identities and credentials are issued, managed, verified, revoked, and audited for authorized devices, users, and processes.” That definition covers multiple control functions that affect risk in very different ways. So if PR.AC-1 was scored as a “2”, does that score represent the most capable aspect of the subcategory, the least capable, or some sort of average? The bottom line is that if all you’re trying to do is roughly gauge and communicate about the overall condition of a cybersecurity program, then using broad brush definitions and an ordinal score is fine. If, however, your objective is to quantify cybersecurity risk in real terms, then you’re out of luck.
And for the record, the second of these problems is true to some degree in the other control framework standards as well. I’ll discuss this in a future blog post regarding what we’re learning as we map these control frameworks to FAIR-CAM.
In the interest of avoiding TL;DR, I’ll wrap up the discussion on data in the next (and final) post of this series, and touch on what is possible from a CRQ automation perspective. I’ll also pull together everything from the series to provide an overall conclusion. Thanks for hanging in there with me.
Read the series by Jack Jones on automating cyber risk quantification.