Jack Jones Rebuts ‘FAIR Fatigue’, an Article Filled with Misrepresentations of Factor Analysis of Information Risk (FAIR), the Standard for Risk Quantification
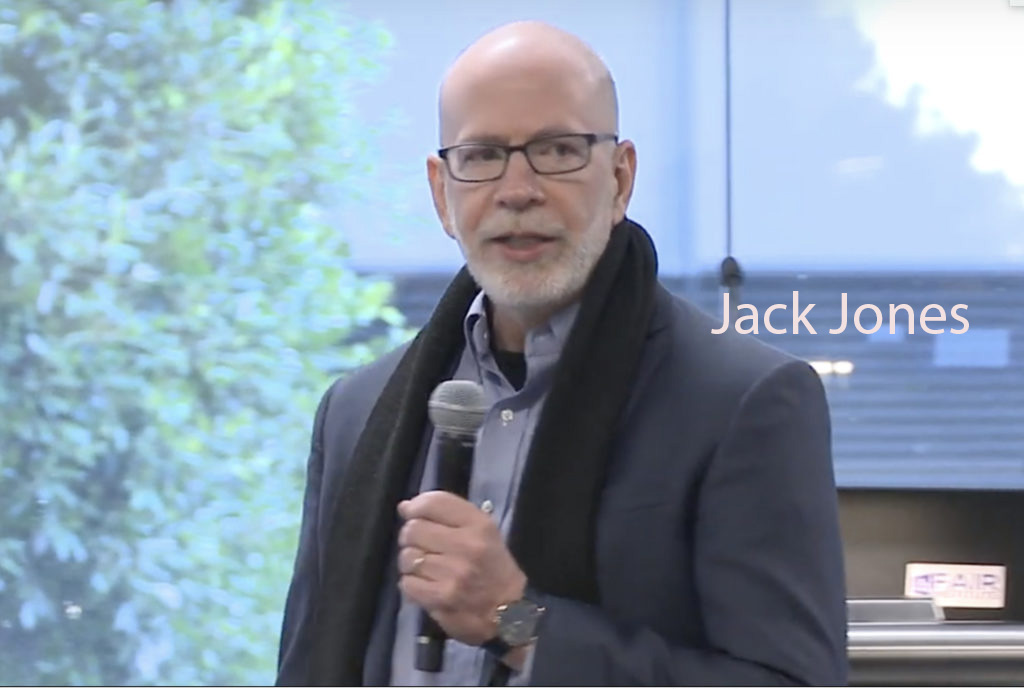
It’s not often that I’m surprised by someone’s actions on the Internet, but I’ll admit to being surprised today. You see, David White wrote an article discussing what he refers to as “FAIR Fatigue.”
I felt his article misrepresented FAIR, especially relative to proprietary risk models, so I wrote a rebuttal comment under his article. Apparently, someone didn’t like what I said and removed my comment.
Now, if any of the statements in my rebuttal were factually inaccurate, all someone would have had to do was point out my error(s) and I’d have issued a retraction. At the very least, I’d have expected a rebuttal to my rebuttal. But no; instead, my rebuttal was removed. I guess alternative points of view and open debate aren’t welcome (I’m a little surprised someone didn’t also remove Evan Wheeler’s comment).
Jack Jones is Chairman of the FAIR Institute and creator of Factor Analysis of Information Risk (FAIR)™, the international standard for quantification of cyber, technology and operational risk.
So, in the interest of setting the record straight, I’ve posted my rebuttal below. Again, if anything I’m saying is factually inaccurate, I’m happy to post a correction. In the meantime, you be the judge.
“FAIR Fatigue” – A Rebuttal
I’m actually glad David wrote this post because it gives me an opportunity to set the record straight on some common misperceptions regarding FAIR, especially relative to proprietary models. Specifically:
“Perfect Data” Isn’t in Demand
David is correct that some organizations have struggled with adopting FAIR, but this isn’t a function of FAIR itself. Rather it’s a function of how some organizations approached its use. If an organization insists on digging for “perfect” data, to remove virtually all uncertainty in their measurements, then yes, they’re more likely to burn themselves out. But most organizations, especially if their analysts have been trained in FAIR and are critical thinkers, don’t fall victim to that. This applies as well to his statement about “over-emphasis on probabilities”.
David forgot to mention that FAIR has a history of solving headaches and improving an organization’s ability to focus on the things that matter most, as well as being a valuable tool for explaining and defending risk analysis results to executives and regulators.
Proprietary Models Can’t Be Explained or Defended
This second point is very difficult, if not impossible, to accomplish with a proprietary model. If an inquisitive executive wants to know how you arrived at your risk measurement, it’s usually important to be able to explain the model, the assumptions, and how data were applied. Having to rely on, “This is what the technology spit out” would be a very uncomfortable position to be in.
Speaking of which, it’s still surprising to me that a profession that recognizes the folly of proprietary encryption would tolerate proprietary risk measurement models. It is profoundly easy to design a fundamentally flawed risk measurement model, resulting in inaccurate measurements and bad risk management decisions. That’s the main reason why FAIR was made an open model, so that the community could poke and prod at it, improving it over time.
And This Claimed ”Uncomplicated Method” Isn’t Explained, Either
David mentions an “uncomplicated method for estimating the core aspects of probability”, with a link to an Axio page that provides absolutely no explanation of how that’s accomplished. It certainly doesn’t provide any way to understand how “significant impacts immediately become apparent.” Then again, as a proprietary model, I guess that shouldn’t surprise me.
“Not sweating the small stuff” is a great approach… when you understand which things qualify as “small stuff” and why they don’t matter. But I guess we’re supposed to take Axio’s word that they’ve worked that out. We certainly don’t have an opportunity to determine for ourselves whether we agree with their assumptions on the matter.
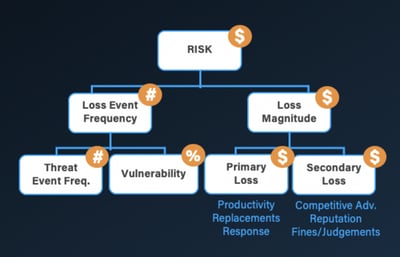
No, FAIR Isn’t about Driving Risk to Zero, Modeling All Impacts or Obsessed with Granularity
“We will never drive cyber event probability to zero; as long as the probability is non-zero, an organization must endure the impact to survive.” I’m not at all sure what that has to do with FAIR, as it’s a fundamental truth regardless of how you measure risk. As for “modeling all categories of impact”, FAIR was the first model to decompose impact, and it’s done so in a way that’s stood the test of time. David doesn’t explain how Axio’s approach is any different or better.
As for Forrester’s report, they’re right about FAIR not being the only approach to CRQ, but it is the only one that’s a non-proprietary industry standard with a professional certification for practitioners, a community of over 13,000 members globally, and that’s included in numerous university programs.
And with regard to FAIR being “obsessed with fine-grained probability”, here again, this statement demonstrates either a lack of understanding or willful mischaracterization. FAIR is perfectly capable of being applied at higher levels of abstraction within the model itself, as well as with less granularity in how analyses are scoped. In fact, this flexibility is one of its notable strengths — you can get into the weeds when necessary and avoid the weeds when it makes sense. This also applies to David’s statement regarding combinatorics.
The Bottom Line…
The bottom line is that David’s piece displays either a remarkable lack of familiarity with (or selective characterization of) how FAIR can and should be used. That said, maybe Axio in fact has developed a really great solution that’s meaningfully better than FAIR. Unfortunately, as a proprietary solution, we’ll never know if that’s the case. We’ll just have to take David’s word for it.