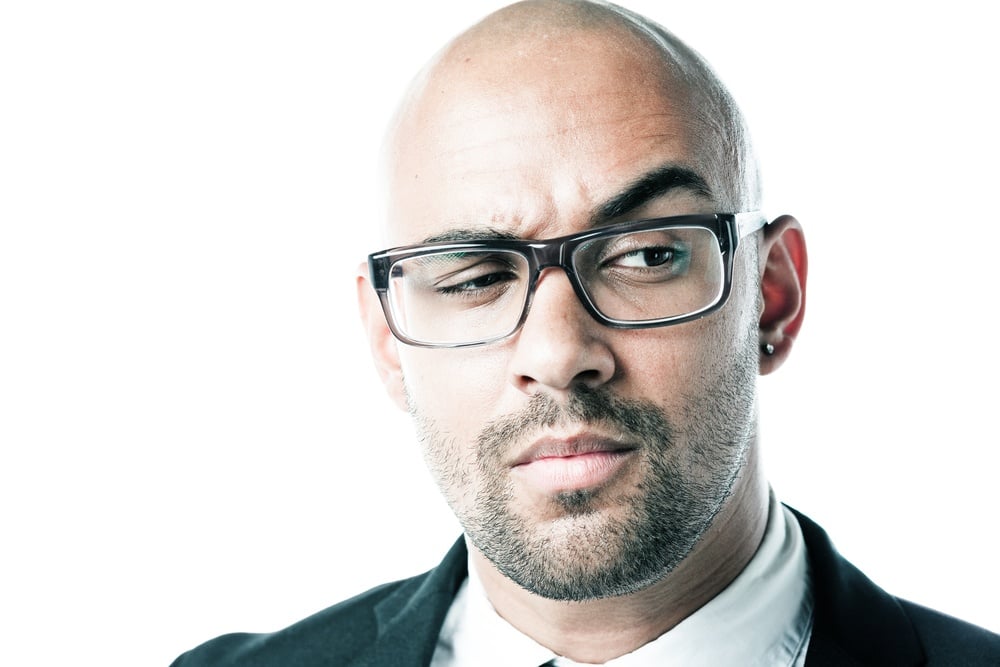
I recently attended the SIRACon conference in Seattle where I had the privilege to hear leaders from prestigious companies speak about their experience using quantitative analysis of cyber risks. One of the presentations that stood out related to sources of error and bias in survey results. The points that were made deeply resonated with me, since part of my job as a risk consultant is to help organizations quantify the results of their risk analyses in terms of dollars and cents which requires – you guessed it –numerical values. One of the sources that organizations leverage when they do not have reliable figures internally is data from industry reports.
The challenge; however, with these reports is that not all surveys are created equal and if you don’t perform the appropriate due diligence upfront, you could end up misinformed. Daniel Levitin says it well in his book, A Field Guide to Lies and Statistics, “just because there’s a number on it, it doesn’t mean that the number was arrived at properly.” This concept directly applies here and encourages analysts before leveraging an industry report to get an understanding of some of the limitations and adjust their confidence in these data points accordingly.
There are a multitude of errors and biases that can enter into the data collection process. These impact not only the risk analysts performing the work, but the consumers of this information, who rely on this data to make decisions and prioritize investments. Although errors cannot be 100% be eradicated, here are a few you can protect against:
Coverage Errors
Description of error: Lack of diversity or unspecified characteristics of individuals in the population included in the sample.
Example of error: The survey method does not explain how the survey was administered.
Improvement: The method used to survey samples from the population should be clearly documented. Additionally, look for surveys that use a mixed methodology approach to data collection, which can assist in maximizing responses from hard-to-reach respondents (i.e., multimode design).
Sampling Errors
Description of error: Individuals included in the sample do not represent the characteristics of the population.
Example of error: The survey selects samples based on convenience. An example may include using a web-based collection method and not accounting for the fact that non-web responses by mailed survey or telephone call would result in a different pattern.
Improvement: Good samples are representative of the population. In other words, every sample should have an equally likely chance of being chosen. Look for survey methods that have clearly identified the population of interest and use diverse participant recruitment strategies or stratified random sampling.
Measurement Errors
Description of error: Questionnaires/interviews do not evoke truthful answers or accurately reflect the topic of interest.
Example of error: Respondents may not understand what’s being asked or may be distracted. They also may provide estimates that are over- or understated.
Improvement: Look for surveys that use valid, reliable instruments. Incorporating pretest questions also helps to ensure respondents are calibrated.
Nonresponse Errors
Description of error: Lack of response from all types of individuals in a sample. This may be due to a difference in those willing to participate. Those who do choose to participate may show bias for or against the survey topic.
Example of error: Surveys are administered to a representative sample of individuals; however, the populations of non-responders are substantially different in terms of underlying benefits from those who completed the survey.
Improvement: Look for survey methods that formally define follow up procedures for non-responders.
You can look for these above items in the section of the report that describes the data gathering approach. If the approach is not called out, you should exercise additional skepticism.
Below are two industry report sources that I prefer to leverage for cyber risk analysis:
- Verizon Data Breach Investigation Report - This report is not based on survey results, but rather, analysis of actual data breaches broken down by industry.
- Cyentia Library Reports - The Cyentia Institute offers a variety of cybersecurity reports that leverage surveys administered via several of the techniques noted above, including calibration.
In summary, analysts should exercise professional skepticism when leveraging industry reports for cyber risk analyses. Additionally, consumers of these risk analyses (CISOs, CIOs, Board of Directors, etc.), should be sure to ask the right questions to ensure their risk analysts have done their homework.
Related:
Ponemon Report on the True Cost of Compliance -- A Missed Opportunity
Factor Analysis of Information Risk (FAIR) is the international standard quantitative model for information security and operational risk. FAIR practitioners apply critical thinking to risk factors and data sources to make accurate – and defensible – estimates of risk in monetary terms.