State of the FAIR Movement: Jack Jones’ Thoughts on His Lifetime Achievement Award
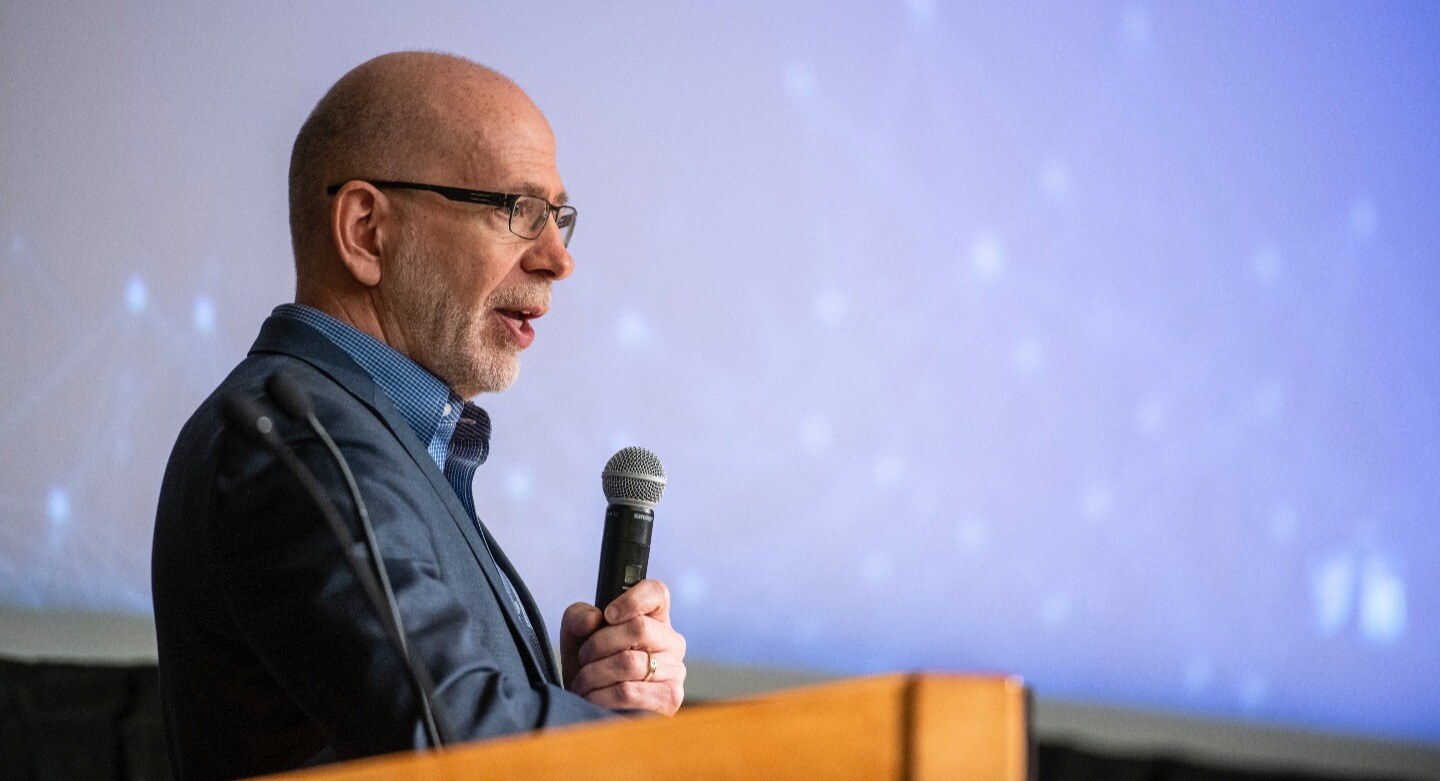
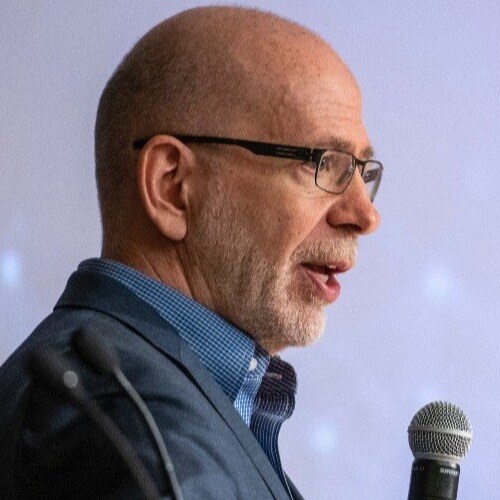
At last week’s FAIRCON 2024, FAIR Institute President Nick Sanna presented the Institute’s Lifetime Achievement Award to Jack Jones, Chairman Emeritus of the FAIR Institute, creator of Factor Analysis of Information Risk (FAIR), co-author of Measuring and Managing Information Risk, a three-time CISO – and a tireless and patient explainer of the need for the cybersecurity profession to adopt cyber risk quantification and a more scientific approach to risk management.
I checked in with Jack for a wide-ranging conversation looking back and forward on FAIR, and how it started with some hand-written notes and evolved into the model for quantitative cyber risk analysis recognized by the National Institute of Standards and Technology.
Q: Congratulations on your Lifetime Achievement Award Jack. What’s your reaction?
A: It’s a very humbling sort of thing and a really nice surprise. My inclination is to think of this as a team award because without being surrounded by so many dedicated people, great ideas, like with a movie, can just end up on the cutting room floor.
Q: I also wonder about your reaction looking out at the crowd at FAIRCON, these hundreds of people who had traveled far to learn about FAIR or celebrate FAIR.
A: I certainly didn't have any of this envisioned when I put FAIR together. I was just trying to be more effective as a CISO. As I developed and applied FAIR I cautiously put it out there and kept getting positive feedback. That encouraged me to share it. Since then, it’s just taken on a life of its own, which is great because one person can’t carry the flag alone for something like this.
This journey has been amazing. It's been uplifting for me to feel like I've contributed to the profession in some way, and it's great to see organizations getting real value from it, and careers being built from it. But it’s still a bit of a shock.
Q: You've told the story about when you were a CISO at Nationwide Insurance meeting with one of the CIOs who set you on your journey to FAIR when he asked you to justify your budget for how much risk you would reduce in financial terms.
A: He’d had run-ins with security in the past and he was going to put me in my place by asking questions he knew I wouldn't have an answer for. But he wasn't counting on me being as persistent as I was.
Q: But at the time you were just following conventional wisdom that cyber risk couldn't be quantified. How did you begin to get your arms around the challenge?
A: Literally, the first step I took was to look up the word ‘risk’ in the dictionary because he was using this term differently than I had been using it; a weak password is a risk and that sort of thing,
And so I went to the dictionary that same afternoon, looked up the word ‘risk’, and the definition that seemed to fit what he was looking for was ‘exposure to loss’.
Exposure I interpreted as the probability or frequency of adverse events and loss as lost magnitude. And so that was the first layer of abstraction in the model and then I thought, what affects the frequency or magnitude of loss and I just kept decomposing it logically. I still have the handwritten notes that I put together over months and years developing this.
Along the way, I had to tell myself to keep to first principles and I read lots of books on probability, decision-making, physics – anything but information security – to provide threads to pull on that were outside my experience. Everything I’d read from information security just took me down dead ends.
Q: What was an early milestone that made you think that you were on the right track?
A: Once I got my team trained and we began reconciling the risks we had to manage, we reduced by an order of magnitude the number of ‘high risk’ issues we were wrestling with – and that was FAIR in its most rudimentary state. It just provided so much clarity. That was huge for us, because it allowed us to focus on the things that really mattered. This also significantly changed how our executive stakeholders perceived us. We were no longer perceived as “crying wolf” all of the time.
Q: Did you set out to make FAIR an open standard?
A: No, not per se. I knew it needed to be widely available, but I hadn’t considered the possibility of it becoming a formal standard. However, not long after publishing my first white paper on FAIR, a couple of senior people from the Open Group reached out to me about making it an open standard – which I thought was a great idea.
Q: However, there's been a proliferation of marketing claims on cyber risk quantification and you've warned people to be skeptical.
A: There's a whole spectrum of providers out there, from those who care very much about strong models and they know what it means to have a strong model. And there are those at the other end of the spectrum, who really don't have a clue but tell a good story. It's inevitable in a new discipline like this. Over time, the market will become better educated and providers who don’t have their act together won’t survive.
In the meantime, it’s vitally important for people to understand what to look for and what questions to ask of providers.
Read more: Jack Jones' CRQ Buyers Guide
Q: Let’s talk about the challenges that FAIR still must overcome. A couple common objections come to mind:
--Cultural inertia: “We don’t need to bother with quantitative cyber risk analysis. It’s too complicated, and besides, what we’ve been doing works just fine.”
--Data: “We don’t have good records on frequency and magnitude of cyber incidents.”
--Scalability and automation – “FAIR analysis is too manual for a large organization.”
A: On the culture question, I equate where we are today to the development and adoption of modern medicine in the 1800s. Bloodletting had been ‘best practice’ for over a thousand years, and here comes this whole notion of bacteria, viruses and physiology, which was not welcomed with open arms by everyone in the profession. Even today you can find people who are adversarial to modern medicine. Similarly, we’ll never have 100% buy-in to FAIR and cyber risk quantification, but we've made remarkable progress. It doesn’t happen overnight.
Q: How about on the data front?
A: We can think of data as falling into three categories; threat data, control data, and loss data. I think the best news we have from a data perspective is in the loss magnitude side of the equation. But that's a silver lining to a very dark cloud; we have that data because the bad guys are beating us up every day. That's incredibly unfortunate, but at least we’re able to leverage the data from these events in our analyses and decision-making.
We also have a lot of control data, but it’s usually not the data we need. For example, many organizations can tell you how many of their systems are up-to-date on patches, but that metric isn’t very useful for risk analysis. Instead, we need to know how often systems become susceptible to malicious code due to missing patches and how long they remain susceptible.
Even then, in order to know how much risk an organization has from deficient patching, we also need to understand the condition of other controls, like EDR, that are relevant to code exploitation attacks. The bottom line is that in order to really get a handle on and apply control data we have to think less superficially about the control landscape. This is where the FAIR-CAM (FAIR Controls Analytics Model) can be extremely helpful. But FAIR-CAM is still very new and not yet fully documented, so progress on the control metrics front is still slow.
For intelligence about the threat landscape, much of the data we need is out there. We just aren't looking at it very closely or thinking about it very deeply. For example, if you ask the average information security professional, “What's the Threat Event Frequency for phishing against your organization?” Almost everyone is going to say “hundreds or thousands of times a day.”
But that’s not accurate. Yes, their secure email gateway might be seeing thousands of spam and phishing emails, but not all spam is phishing and probably a majority of the phishing attacks aren't actually attacking the organization. They're attacking individuals with the intent of getting someone’s bank account number. So, this significantly reduces the number of phishing attacks an organization faces. Then we also need to recognize that most attacks against organizations take the form of campaigns, with each campaign being comprised of multiple phishing emails.
The key here is that the actual phishing threat event frequency isn’t based on the number of emails but rather the number of campaigns, which is a much smaller number. We have similar problems with other threat-related data like attacks against websites. Until we start thinking a little more deeply and clearly like this, we can’t make good use of the threat data we have.
Q: Where do you see the FAIR movement going from here?
A: Eventually, cyber risk quantification, whether it's FAIR or some other approach, is going to be considered best practice in the industry and if an organization isn't doing it then they're going to be considered deficient. Regulation and the influence of the Big Four consulting firms will also help push the industry in this direction. For it to become ubiquitous though, you need solutions that are reasonably easy to apply and scale. And that's where automation comes in, but automation is dependent on data and we just talked about some of the challenges with data. Regardless, that's the direction it's headed.
Read more: Jack Jones on Automating Cyber Risks Quantification
Q: How about AI - is FAIR proving to be adaptable?
A: One of the nice things about FAIR is, it's agnostic. You can apply it to any sort of adverse scenario, whether it’s poor decision-making due to a poisoned or biased AI model, or AI hallucinations, or any other AI-related adverse scenario. You just have to carefully scope the scenario and think about all the things you need for a typical FAIR analysis.
There’s also huge potential for applying AI to FAIR analysis. Unfortunately, AI is only as good as its training and for training you need data, and outside of the loss magnitude side of the equation we’re simply not there yet. That said, large language models can be a very effective addition to risk analysis user interfaces and for presenting an organization’s risk data.
Learn FAIR, meet FAIR practitioners - Join the FAIR Institute.