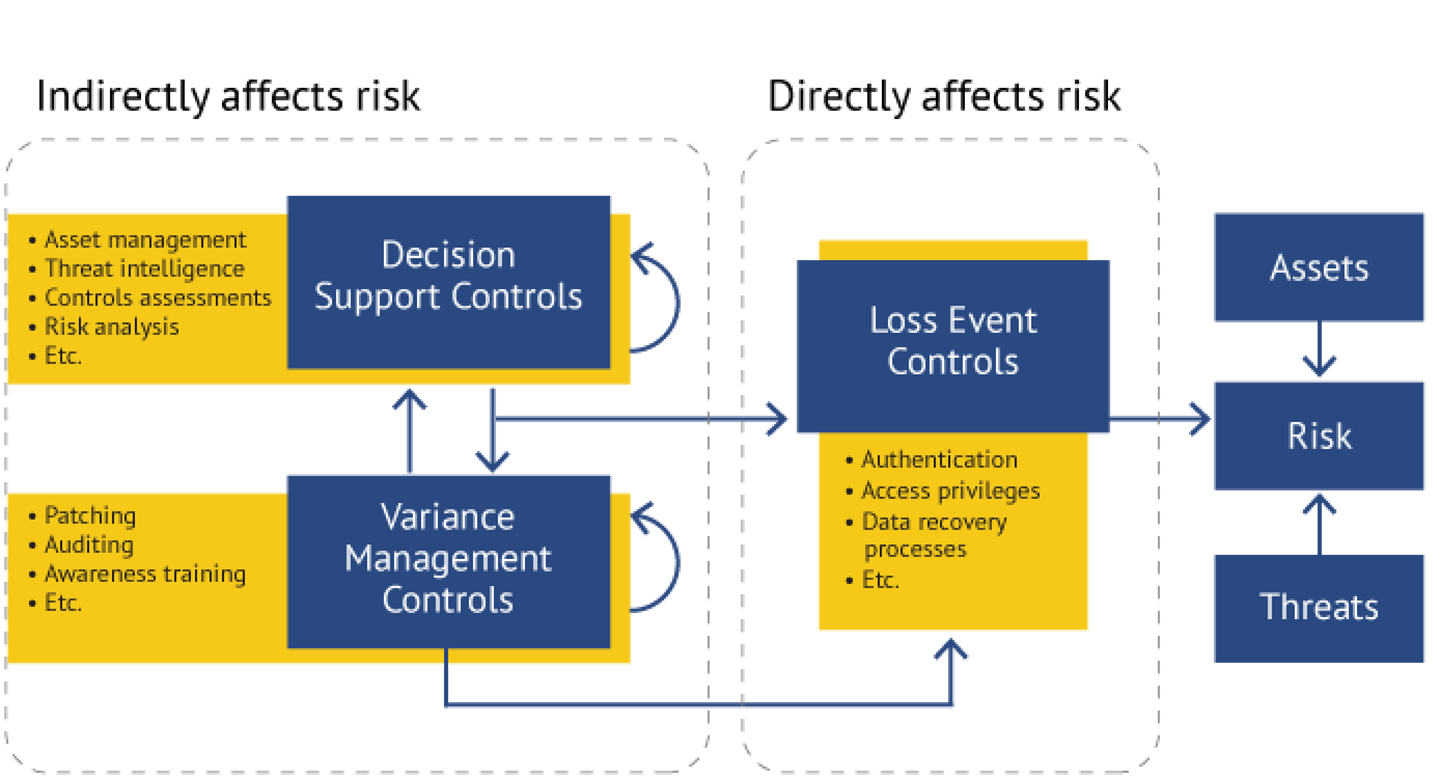
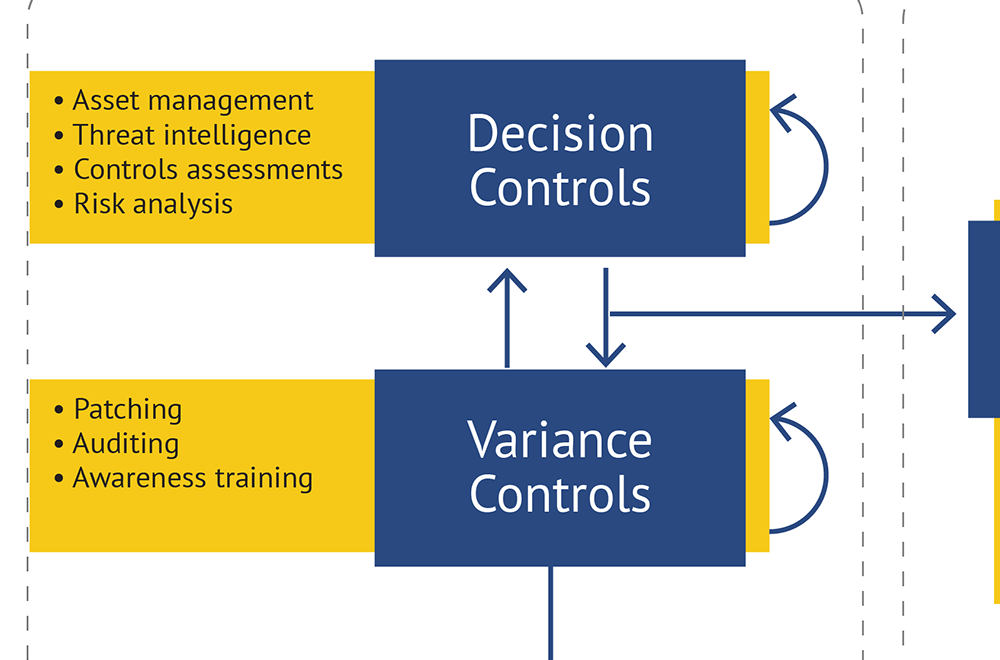
Satya Nadella (Microsoft Chairman and CEO) and Marc Benioff (Chair & CEO at Salesforce) declared 2025 as the year of Agentic AI. While the autonomous decision-making characteristics of an AI Agent unleashes its creativity, it also renders these decisions to be unpredictable.
In this blog post, we introduce the FAIR-CAM “controls physiology”standard and show how to apply the model to understand the risk dynamics between AI Agents and their human users. We will discuss an Open Banking use case to demonstrate this dynamic risk modelling approach.
Denny Wan is Co-Chair of the Sydney Chapter of the FAIR Institute, a member of the Standards and TPRM Work Groups, CI-ISAC Australia Ambassador for Cyber Threat-Led/Informed Risk Measurement, and host of the Reasonable Security podcast.
Operationalizing the FAIR Framework for Cyber Risk Management
As cybersecurity leaders face an increasingly complex risk landscape where the stakes—financial, operational, and regulatory—continue to climb, there is an urgency to concretely understand the causal effects between controls and consistently measure the expected financial losses.
The FAIR Framework – including the FAIR™ Model, FAIR Controls Analytics Model (FAIR-CAM™), and FAIR Materiality Assessment Model (FAIR-MAM™) – is a blueprint for addressing these business challenges. Together, these standards enable organizations to assess cyber risks in financial terms, evaluate the effectiveness of controls, and analyze potential losses with greater precision and reliability.
The FAIR Model serves as the foundational structure by breaking down risk into Loss Event Frequency and Loss Magnitude, while FAIR-CAM builds on this by detailing the impact of controls on risk factors. FAIR-MAM adds depth by providing a detailed taxonomy for analyzing Loss Magnitude and aligning risk management practices with regulatory requirements and organizational priorities.
A Cyber Risk Management System (CRMS) is fundamental for operationalizing these risk management processes.
The FAIR Framework architecture
What Is Agentic AI?
Nadella and Benioff drew attention to the rise of Agentic AI in their recent podcasts, “Satya Nadella | BG2 w/ Bill Gurley & Brad Gerstner” and “Why Salesforce Isn't Hiring Software Engineers” respectively. Marc explained that his total engineering team will grow through the deployment of AI Agent software engineers without hiring additional human software engineers. In fact, Marc has doubled down his effort in the AgentForce World Tour globally since November, 2024.
His view is similar to Satya who believes the SaaS (Software as a Service) format will be replaced by AI Agents. Unlike SaaS which has defined features and user interface, AI Agents can be customized for any workflow without imposing on business workflows to fit in with the SaaS design.
Agentic AI takes advantage of the analytical power of a GenAI Large Language Model (LLM) to adapt to changing environments and events. AI Agents optimize decision-making processes according to the business rules encoded in them.
The recently released OpenAI Operator preview is OpenAI’s first AI Agent platform which harnesses the intelligence of its ChatGPT (LLM) and O1/O3 reasoning models. Operator is designed to “operate” on behalf of their human users based on the recommendations produced by their GenAI counterparts. It relieves the administrative burden of their human users, for instance, in coordinating and aligning multiple decisions such as flights, accommodation, local activities and restaurant bookings to fit with a travel plan and budget.
Without Operator, some of these recommendations might have already been booked by the time the reservation is made in the suggested sequence forcing a change of plan. An AI Agent can initiate and change multiple bookings in parallel, minimizing the likelihood of forced change in plans.
In other words, AI Agents rely on patterns and likelihoods to make decisions and take actions, as opposed to deterministic systems—such as Robotic Process Automation (RPA)—that follow fixed rules with predefined outcomes. Agentic AI now makes it possible to automate many workflows and business processes that deterministic systems have not been capable of addressing on their own.
Herding Cats – Modelling Agentic AI Risk with FAIR-CAM
While the promise from Agentic AI of enabled automation is exciting, the non-deterministic nature of these autonomous decision processes demand a new approach to model the operational risks. Managing a fleet of AI Agents could feel like herding cats which have minds of their own. They do not pay much attention to their owners beyond their biological rules programmed in their genes. These dynamic relationships are similar to the physiology of organs in our bodies which also function autonomously. The groundbreaking FAIR-CAM standard for “Controls Physiology” is perfect for modelling Agentic AI risks.
FAIR-CAM is comprised of three control domains as depicted below:
FAIR-CAM builds on the strength of FAIR to measure the efficacy of controls. For example, the Loss Event Controls (LEC) identify control settings which reduce the likelihood of Loss Events (LEF) and accelerate the containment of an incident to reduce Loss Magnitude. Annualized loss exposure (ALE) is expected to increase when the efficacy of LECs deteriorates.
The Variance Management Controls (VMC) are the cornerstone for modelling the “physiology” of controls through the Prevention, Identification and Correction of variances. The autonomous functioning of human organs can be modelled through the VMC lens.
Changes introduce variance. When people’s lifestyle changes, perhaps due to changing diet, workload or even weather, the body and its organs respond to the changes. While an “unhealthy” lifestyle change (such as overeating to deal with stress) deteriorates the physiology of the body, equally a “healthy” lifestyle change (such as exercise and eating fresh fruit) can do wonders in improving the state of physiology.
VMC is the lens to detect when and where variance occurs to inform options to correct the variance condition and, if appropriate, prevent future variances from occurring. VMC is well suited to model Agentic AI risk by detecting when and where variance in the autonomous decisions were made by AI Agents.
Because AI Agents make autonomous decisions by taking into consideration a number of inputs, including insights and recommendations made by GenAI, there is no hard-and-fast rule to declare a variance condition has occurred.
Just because there is an opinion that disagrees with the decision, it is not a sufficient condition to declare a variance condition. Measuring the efficacy of VMC is a more comprehensive and consistent way to detect a variance condition. This approach is foundational to the modelling of Agentic AI risks.
The Decision Support Controls (DSC) indirectly affect risk by enabling organizations to make decisions that are aligned with the expectations and objectives of leadership. This control employs the most complex ontology structure in FAIR-CAM, drawing in asset data, threat data and control data. Expectations and objectives of leadership are often expressed in high level financial terms such as earning targets, profit margins and risk appetite statements. Measuring the changes in the efficacy of DSC is a useful yardstick to inform the trajectory of the direction of the decisions made.
The above analysis illustrates the difficulty in modelling human physiology or Agentic AI risk due to the non-deterministic nature of both control classes. There is no right or wrong control outcome because “it depends” on perception of a “good outcome”. It is more reliable to measure the overall impact from these collections of non-deterministic controls such as the lack of serious health issues or major financial loss.
Open Banking Use Case
Open Banking is set to disrupt the financial services industry by pushing credit decisions to the edge of the financial services supply chain. Buy Now Pay Later (BNPL) showed the market opportunity in pushing credit decisions to the edge, at the point of purchase. BNPL was promoted as a payment instrument (similar to PayPal) instead of a credit product. Therefore, the BNPL credit approval algorithm did not include credit worthiness verification of the consumers in the past.
This practice has shown to be causing consumers to get into financial difficulty. Financial regulators have been tightening up this loophole pushing BNPL providers to comply with consumer credit laws to protect consumers from getting into financial difficulties. These additional regulatory compliance checks are difficult to perform in real time at the point of purchase, limited by access to the consumer’s financial information such as income, current debt obligations and payment default histories.
Adding AI Agents to the BNPL technology stack could ease the technology cost in delivering real time consumer credit scores by taking advantage of the Open Banking infrastructure and insights generated from associated GenAI trained data sets. These AI Agents are more capable of implementing and enforcing financial security regulations such as Anti-Money Laundering (AML) in addition to calculating FICO credit scores.
The innovation of the BNPL business model is to calculate the risk of consumer payment defaulting based on the type of goods being sold, sales channel, location of the merchant (brick and mortar or online) and time of the purchase. For example, a higher credit amount is more likely to be approved for purchasing groceries in a supermarket during the daytime than purchasing cigarettes or alcohol late at night in a convenience store.
While GenAI can be pre-trained with the consumer’s credit history data set, the AI Agent is needed to capture real time consent from the consumer for their data to be used for this credit scoring purpose for a particular transaction.
The AI Agent embedded into the checkout flow is also better placed to confirm the physical location of the consumer and merchant, to minimize the risk of payment fraud committed by bots masquerading as legitimate merchants.
These additional checks would be performed by interacting with other AI Agents in the merchant store such as the checkout scanners and POS payment terminals. Each of these agents will make its decision independently given its own set of business rules. The POS Payment terminal, for example, will need to verify the assurance level and trustworthiness of the authorization of the payment approval presented by the BNPL AI Agent. The FAIR-CAM “Controls Physiology” model is best suited to model the interaction between these complex sets of autonomous decisions.
Conclusion
Agentic AI, drawing on inference from trained GenAI datasets, will open up new frontiers and possibilities in many global supply chains. It reduces the constraint in these supply chains by distrusting the trust and decision points.
For example, the global credit card payment system (Visa/MasterCard/Amex, etc) has been the default for a universal credit approval infrastructure allowing travellers to complete credit transactions anywhere in the world where these credit cards are accepted.
Agentic AI technology leveraging Open Banking technology holds the promise of distributing financial credits outside the card network at lower cost to the consumers and merchants. A reliable and scalable risk modelling framework, such as FAIR-CAM, which understands the interplay of “Controls Physiology” between these AI Agents, is the foundation to unlock these potentials.
Please join the FAIR Institute to learn more about the FAIR-CAM standard and the FAIR Framework.