Why Risk Teams Should Be Champions for Data Governance in Fintech Firms
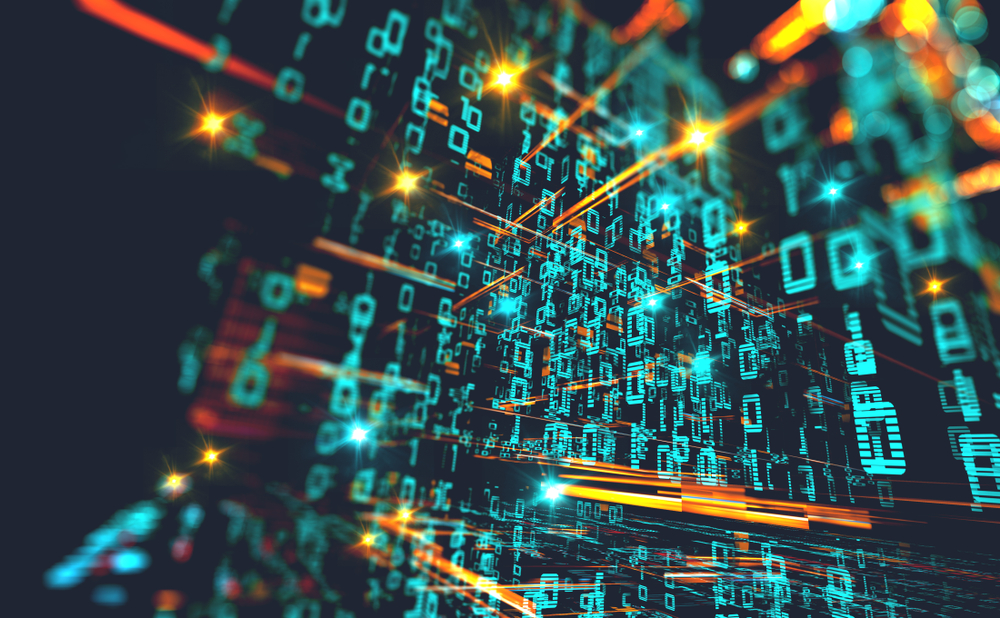
Ask a cyber risk professional about data governance practices, and they will likely tell you tales of classification schemes, access controls, and encryption … but we often overlook the importance of data quality, integrity, and usability that are core tenants of a robust data governance process.
As risk professionals, we rely heavily on data that is sourced both throughout our organization and from industry partners. No matter how sophisticated your analysis methodology is, garbage data in often means garbage results coming out.
Fintech firms, especially, can be caught in the push-pull of needing to be light and nimble to get ahead of the entrenched financial firms and at the same time having high regulatory demands that are data heavy. Often investments in data governance structures are deferred or entirely overlooked by start-ups. However, it is a business reality that data is truly the lifeblood of all Fintech firms, and it can be a costly mistake to delay implementing some basic data governance practices early on.
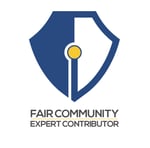
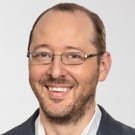
Risk Teams: Question the Quality of Your Data
To put it into perspective, the Chair of a Fintech company’s Board Risk Committee was once asked in a town hall meeting if he worried about the risks of an ongoing migration of their core systems into the cloud, and he responded that he was far more worried that the majority of the strategic decisions made in the company are based on spreadsheets with no quality controls or validation. Let us not forget that data quality needs to have equal priority to security and privacy when it comes to data governance.
Risk teams are in the ideal position not only to shine a light on the data governance gaps, and how those can severely hinder well informed decision-making, but they should also be an example to the rest of the company for what it looks like to implement robust data governance practices by applying these principles to their own risk programs first.
Think about every KRI on your reports to senior leaders – do you know where the supporting data is sourced from, are automated validation checks in place to flag anomalies, and would you know in advance if the data sources were changed or decommissioned?
Let’s take a simple example, assume that you’re tracking the percentage of company owned laptops that are encrypted and the endpoint security tool’s dashboard reports that agents are deployed to 1,800 laptops and that all 1,800 laptops have full disk encryption enabled.
Seems like good news, but wait, how do you know that the company only has 1,800 active laptops? Has this been compared to an asset inventory? What about the decommissioned laptops sitting in a closet waiting to be wiped or destroyed? In this case, measuring control coverage is closely related to the concept of data completeness, which is just one element of data quality.
In some ways this is a good news / bad news story. The good news is that you have automated a bunch of the data inputs into your risk program – take a minute to savor this success. Now put the champagne down and let it sink in that you are entirely dependent on all these data sources, data pipelines, and reporting tools which you likely don’t directly control.
If data is missing, inaccurate, or simply misinterpreted, then your program could be causing the organization to make some really poor decisions. Anyone who has experienced the embarrassment of having to report back to a risk committee or board that the last month’s numbers were materially “off” will do anything to avoid being in that position a second time.
Learn FAIR quantitative risk analysis through the FAIR Institute. Take the FAIR Analysis Fundamentals course.
Checklist for Data Governance Controls
Oversight of data quality is a logical starting point, but ultimately, we care about information quality for decision-making. Even with the highest quality data, we can lose information quality if the data isn’t relevant or is misinterpreted. Data governance controls should cover:
- Data – controls that help to understand the accuracy, completeness and timeliness of raw data used for analysis
- Analysis – controls that help to ensure that data are correctly interpreted and turned into accurate insights
- Reporting – controls that provide useful analysis results to stakeholders in time to support decision-making
Exception tests and regression tests should be run regularly to minimize the likelihood of errors being introduced during any transformation:
- Exception tests look for prohibited data relationships, and set thresholds for expected data types or ranges (e.g., you have ten times as many laptops as employees, or a laptop is associated with more than one employee).
- Regression tests examine data assets before/after code changes.
Any tests that were useful during QA testing of the data would great candidates to be automated and applied to dataset updates before they’re ingested into your systems.
Relevance of Data Quality to Risk Management
Clearly the discipline of risk analysis is highly dependent on data, and the most mature programs strive to be even more data-driven and evidence-based.
But this comes with an obligation to scrutinize the data and implement controls to quickly identify anomalies or inconsistencies in the data feeds. In fact, poor quality data isn’t even necessarily a problem as long as we can recognize it, account for the lower confidence in our modeling, and reflect it in our reporting.
Thinking about data from a FAIR lens, every factor in the ontology is prone to data quality issues, and the considerations will vary depending on the source of the data and how it is being used. Let’s touch on a few examples.
Loss Magnitude
Creating reusable loss tables is a staple of a risk analysis function, but over time we can easily lose the traceability for the source of these ranges and the quality may degrade.
For example, assume you have a range of potential PCI violation fines as “$5,000 to $100,000 a month, depending on factors like the size of your business and the length and degree of your non-compliance.” You’ve been happily applying this range to your scenarios involving breaches of credit card data, but wait, this data was gathered from an accounting firm’s report back in 2017 when you first kicked off your risk program, and hasn’t been updated since.
So many questions come to mind: 1) was the source credible in the first place, 2) was this based on data of similar firms to yours, 3) has anything significantly changed in the enforcement landscape that these ranges need to be updated, etc.
It is so critical that you capture the source of the data being used in your loss tables, and maintain a basic change log. You’ll need to consider what is an appropriate trigger or frequency to prompt updating the loss tables, and also how to update your previous scenario assessments if the range changes significantly.
Another input to your loss tables may be your own internal incident data. This may or may not be captured in a system over which you have direct control, and likely isn’t managed by a process that you own. What if the incident management team (or another risk team) changes the definition for what is classified as an incident, or decides to stop tracking incidents with an impact below a certain threshold – would you know and be able to account for this in your loss tables?
Loss Event Frequency
By far, the largest volume and the most frequently sourced data is related to this branch of the FAIR ontology. By now the potential issues are likely clear, but let’s discuss a few examples to reinforce the points about data quality.
For example, you might be leveraging a threat profile for a hacker group that was developed by another team – you would want to apply the same scrutiny that we discussed previously for the industry reports feeding the loss tables. Take time to validate that the data being provided is credible and also that it is relevant to your risk scenario. Don’t assume that someone who is analyzing threat groups will be familiar with your use case for a threat’s motivation or capability. Explicitly ask them to rate their confidence level. Think about how this will be maintained and updated.
Let’s assume that you’re measuring the effectiveness of a control – this could be through observation of its operation during normal conditions or through some kind of simulated testing or sampling. This could involve sourcing data from multiple different security tools, system logs, and asset inventories just to measure the effectiveness of one control. All these sources need to be in sync and cross-checked for integrity.
Too often we’re just so thrilled to get access to the data in the first place, we don’t follow a thorough vetting process before incorporating it into our assessments. Clearly the degree of QA testing and ongoing validation recommended here isn’t scalable manually, and automation is key.
A lot of time is spent discussing the reliability of subject-matter expert sourced data, and it is easy to overlook the issues that can arise from the many other data sources (automated and not) that we rely on for our assessments and metrics.
Risk’s Role in Oversight of Data Governance
The Risk team can play a central role in sponsoring a data governance program. In many Fintech organizations, the Risk team is well positioned to initially set the standards for:
- Understanding the usage of data in the business
- Setting and enforcing policies and standards
- Creating clear and unambiguous definitions of data, and reconciling conflicting definitions
- Defining roles & responsibilities, and sponsoring training
- Monitoring data quality and sponsoring root-cause investigations when problems arise
Start by applying these standards to the data that the Risk team analyzes and reports on regularly, and set the example for the rest of the organization. As the value of the program becomes clear to the business, the Risk team can help identify critical business processes and top risks that should be high priorities for data governance.
The Future of Data Governance: Collaboration between Business and Technology
Data is flowing through our systems every millisecond of every day, and in and out of our environments just as often. We know the threats to this data are real … according to the Cyentia Information Risk Insights Study in 2020, the information and financial sectors have the second and third largest probability of suffering a publicly disclosable event, respectively. That puts Fintech firms at the cross-section of the most likely sectors to be breached.
Of course, we want to control access to sensitive datasets and limit exposure, but we also need to shift our culture to be thinking about how we enable data sharing in a responsible manner. Instead of the “need to know” and “least privilege” principles, let’s recognize that data exists to be shared.
We should be thinking about the value of data (or more precisely the value of “information” that the data supports) in terms of its role in decision-making. A good data governance program can shape a Fintech firm’s decision-making process, but it isn’t a small undertaking.
It's a cultural shift that requires both business and technology sides of the organization to come together to define data elements and the rules that will govern this data across the enterprise.
Learn More about Data Governance:
If you’d like to learn more about data governance practices and how to get started, the FAIR Institute has published the “Data Governance Practices for Cyber Risk Management” paper by Evan Wheeler which expands on these concepts.