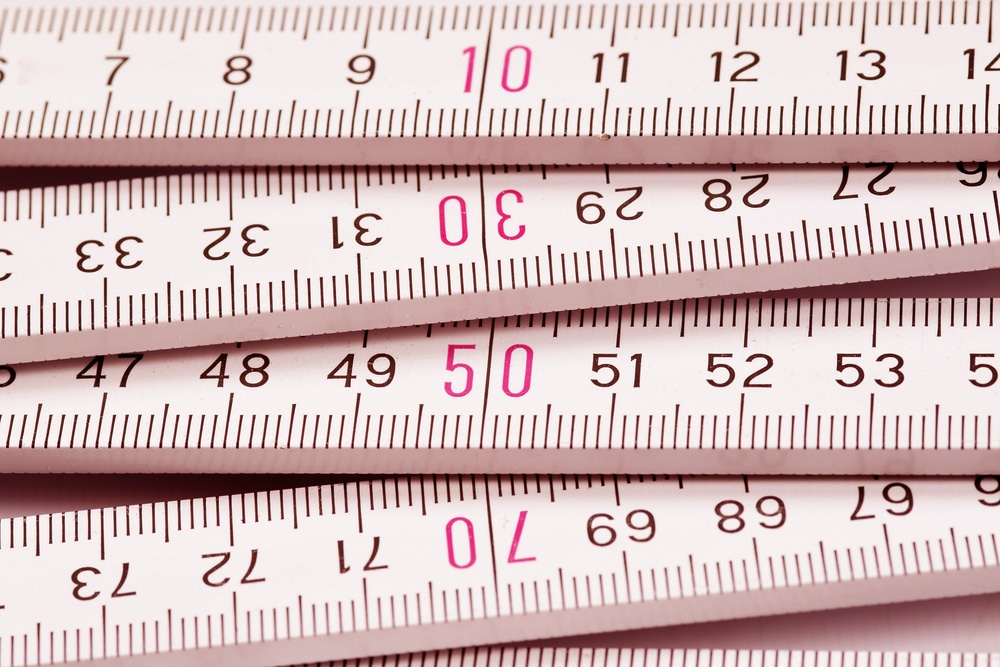
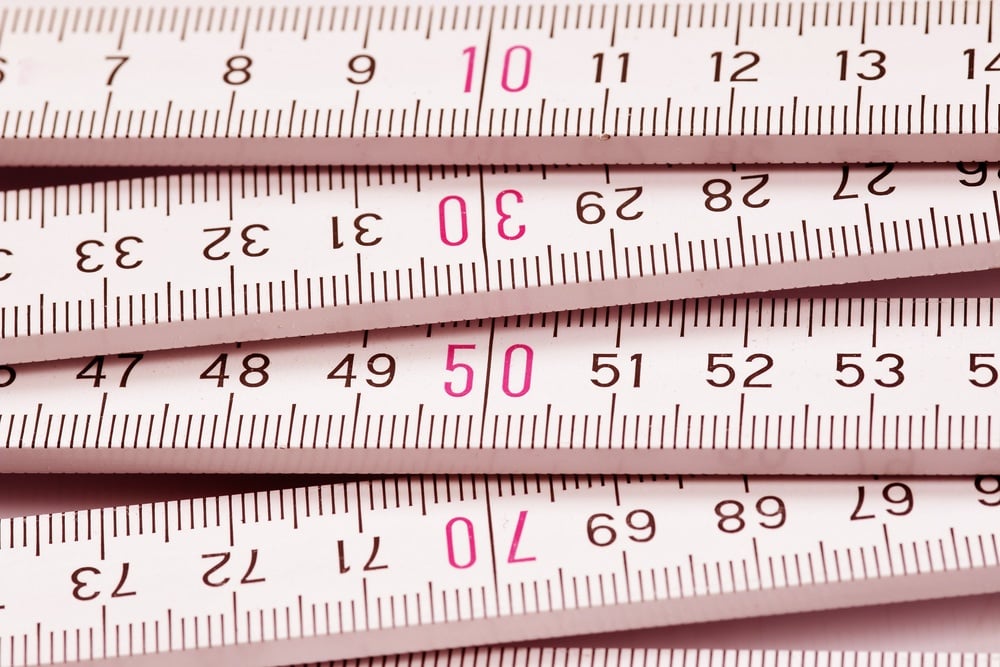
It’s a common misconception about quantitative risk analysis that not “enough” data or“bad” data means bad calibration. That’s not true in a couple of ways. First, one always has “enough” data to conduct an analysis and second, with calibrated estimation, we’re not dependent on the amount of data we bring to the table.
What Is Calibration?
According to Jack Jones and Jack Freund’s book Measuring and Managing Information Risk: A FAIR Approach, calibration is “a method for gauging and improving a person’s ability to estimate effectively.”
The 4 Steps in Calibrated Estimation
-
Start with the absurd
-
Eliminate highly unlikely values
-
Reference what you know to narrow the range
-
Use the equivalent bet method to gauge your confidence level, till you hit 90% confidence.
Get the details on calibration in this blog post:
Calibrated Estimation for FAIR™ Cyber Risk Quantitative Analysis - Explained in 3 to 4 Minutes
In order to calibrate effectively, we need to clearly articulate the scope of the analysis and any assumptions we are making about it as well as the specific data points we are estimating. The amount or type of data we bring to our analysis is independent of our ability to calibrate.
It’s crucial to have the assumptions well documented as they will be a guiding factor in our process of estimating. An inaccurate calibrated estimate (i.e. the correct answer does not fall in your range) is likely less a reflection of your ability to calibrate and more a reflection of your underlying assumptions.
This tells us that we can have well-calibrated estimates based on little data or well-calibrated estimates based on a large data set. The measure of how effective calibration is, is how closely the resulting range matches our 90% confidence interval (i.e. your results are within your range) given the information we have available, our clearly defined scope and our well documented assumptions.
This brings us to the toughest misconception to get past, knowing whether or not an analyst has “enough” data.
What Is “Enough” Data?
Douglas Hubbard, author of How to Measure Anything in Cybersecurity put it perfectly “if you know almost nothing, almost anything will tell you something”.
Douglas Hubbard spoke at the 2019 FAIR Conference. Watch the video.
Our own biases drive the thought that if we don’t collect x amount of data, then we don’t have enough data and can’t conduct our analysis. However, no matter if your data collection efforts resulted in a few data points or a large amount of data points, you will always have enough data. In other words, your first data point reduces your uncertainty more than your tenth and at the end of the day all we’re trying to do when measuring risk is reduce our uncertainty.
The degree to which we need to reduce our uncertainty in order to make an effective decision is what we call the “useful degree of precision”. This varies by analysis, but the guiding principle should be that it is better to be accurate than it is to be overly precise, especially in cases when information is limited. This means that we may not have a large data set but even with limited data, we still have enough to conduct an analysis and calibrate on.
We do this by using the data available to us which is reliant on the analyst’s ability to collect information through industry data or within the organization. Imagine if you need phishing click rate numbers and you know your Security Operations Center (SOC) keeps track of that. Wouldn’t be easy to pick up the phone, send an email and ask for the data? Of course, but it’s key to remember that it’s attainable if those friendly organizational relationships are built so that our peers are happier sharing information with us. That’s why when calibration is discussed, we refer to an analyst calibrating on available information.
Putting It All Together
Here’s a brief example of what this all means when discussing analysts always having “enough” data to calibrate on. Let’s say you’re attempting to determine the threat event frequency, or TEF, for an analysis and your first thought is looking at the phishing click rate for your organization.
However, you quickly find out your organization doesn’t track the phishing click rate for the company; but it does track other data, like if there has ever been a successful phishing attempt. You can also look to industry data on phishing.
Once we collect what is available to us for reducing uncertainty, we begin calibration. Your grasp will differ based on what you’ve chosen to track and measure, the degree to which your team is networked with other data sources, the friendly relationships you build with others in your company so that they’re happier sharing information with you, the degree to which you’re aware of industry publications, etc.
At the end of the day, analysts will always have enough data to calibrate and conduct an analysis if they clearly articulate their scope and document their assumptions. One key thing to remember if you’re still unsure about calibrating: It’s a skill that we all learned how to do, making decisions in daily life. A great resource to look to, to assist in gaining a better understanding of calibration is Douglas Hubbard’s book How to Measure Anything in Cybersecurity Risk. Hubbard discusses in his book how to better measure risk and formulate a more robust risk management program.