FAIR Cyber Risk Analysis for AI Part 3: Identifying and Exploiting Vulnerabilities
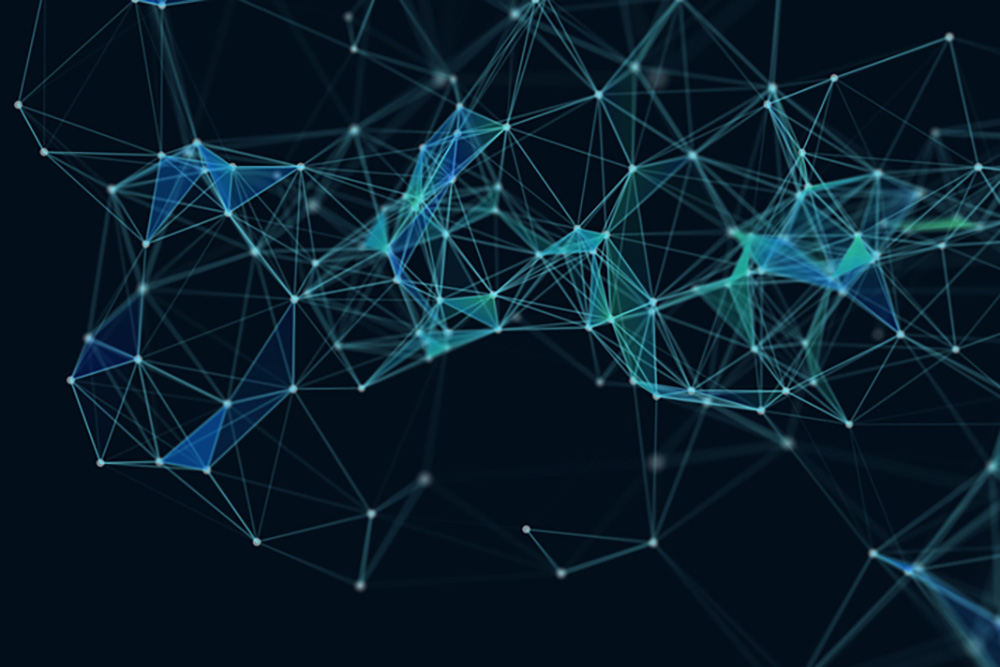
Artificial Intelligence (AI) is a double-edged sword. While it offers exciting benefits and opportunities for innovation, it also presents new challenges and risks, particularly in the realm of cybersecurity. In this blog post series, we continue to explore how AI is transforming the cyber threat landscape and how to quantify resulting loss metrics using Factor Analysis of Information Risk (FAIR). This post focuses on how AI lowers the barrier to entry for malicious external actors to identify and exploit vulnerabilities.
Michael Smilanich is a Risk Consultant and Kevin Gust a Professional Services Manager for RiskLens.
An Example: Cybercriminal Identifies and Exploits Vulnerabilities Using AI Tools
“The Malicious Use of Artificial Intelligence,” a report drafted by 26 authors from 14 institutions spanning academia, civil society, and industry, concluded that AI tools can automate tasks that would ordinarily require intensive human labor, intelligence, and expertise, such as identifying vulnerabilities and developing exploitative code, enabling less technically skilled individuals to launch sophisticated attacks.
For instance, AI-powered tools can scan vast networks to identify potential vulnerabilities in a fraction of the time it would take a human. Once these vulnerabilities are identified, AI can also automate the process of crafting and deploying exploits. This means that attacks can be launched more quickly, and on a larger scale, than ever before.
A recent report from Checkpoint, an Israeli security firm, shares an example of an anonymous user of a popular underground hacking forum sharing their positive experiences utilizing OpenAI’s ChatGPT “to recreate malware strains and techniques described in research publications.”
To demonstrate the capabilities of AI-based malware, HYAS Labs built a proof of concept exploiting a LLM and found that this kind of malware can be “virtually undetectable by today’s predictive security solutions.” Not only can this be created from scratch as HYAS demonstrated, but recent studies have also shown how a threat actor can alter malware into new highly evasive polymorphic mutations using ChatGPT.
Webinar on AI Risk
Attend a webinar: Quantifying AI Cyber Risk in Financial Terms, hosted by RiskLens, Tuesday, June 20, 2023, at 2 PM EDT.
Framing a Loss Event Scenario for FAIR Cyber Risk Analysis: AI-Enhanced Vulnerability Exploitation for Ransomware
To frame this type of incident in a way that allows us to quantify the potential loss exposure, we use FAIR scoping principles to identify a risk scenario:
- Threat: Malicious External Actor
- Effect: Loss of Availability (i.e., service disruption)
- Asset: Network Infrastructure
- Method: Ransomware (developed and/or optimized via AI tools)
To estimate Threat Event Frequency, we might consider:
- How many times has ransomware been detected on endpoints in the past? (i.e., via phishing or downloaded via browser)
- Has there been an increase in the frequency of such attacks since the explosive adoption of AI-powered tools such as Large Language Models (LLMs)?
Next, we estimate Vulnerability (or Susceptibility) by evaluating:
- Controls in place to detect and prevent ransomware propagation on endpoints and to control access to the asset such as:
- Anti-virus software:
installed on endpoints and signatures kept up-to-date?
vendor’s capability to identify and react to emerging threats?
- Anti-virus software:
- Least Privilege: Is access to network infrastructure restricted to only those personnel who require it to perform their job functions? (Sources may include periodic access reviews and audits of provisioning and de-provisioning processes)
- Patching: How effectively does the organization identify and patch vulnerabilities?
- Asset Segmentation: Is the in-scope asset segmented from the rest of the network in a way that could prevent ransomware from propagating to the asset?
RiskLens in-platform guidance (click for bigger image)
Loss magnitude can be estimated by considering costs such as:
- Revenue loss due to downtime
- Incident response: What measures are in place to respond to ransomware attempts
- Reputational damages (i.e., lost customers as a result of downtime)
- Downtime (i.e., loss of employee productivity)
- Potential contractual fines, such as breached SLAs or legal expenses
Conclusion: Navigating the AI-Powered Threat Landscape
The advent of AI has lowered the barrier to entry for malicious external actors to identify and exploit vulnerabilities, adding a new layer of complexity to the cybersecurity landscape. These risks necessitate a comprehensive, risk-informed approach to AI governance. Robust risk quantification models like FAIR, powered by tools like RiskLens, can guide us in navigating this rapidly changing threat landscape. Stay tuned for more posts like this as we strive to stay on top of emerging related cyber risks and discuss approaches to quantifying their impacts
Read our blog post series on FAIR risk analysis for AI and the new threat landscape
Attend the Webinar: Quantifying AI Cyber Risk in Financial Terms, Tuesday, June 20, 2023 at 2 PM EDT.