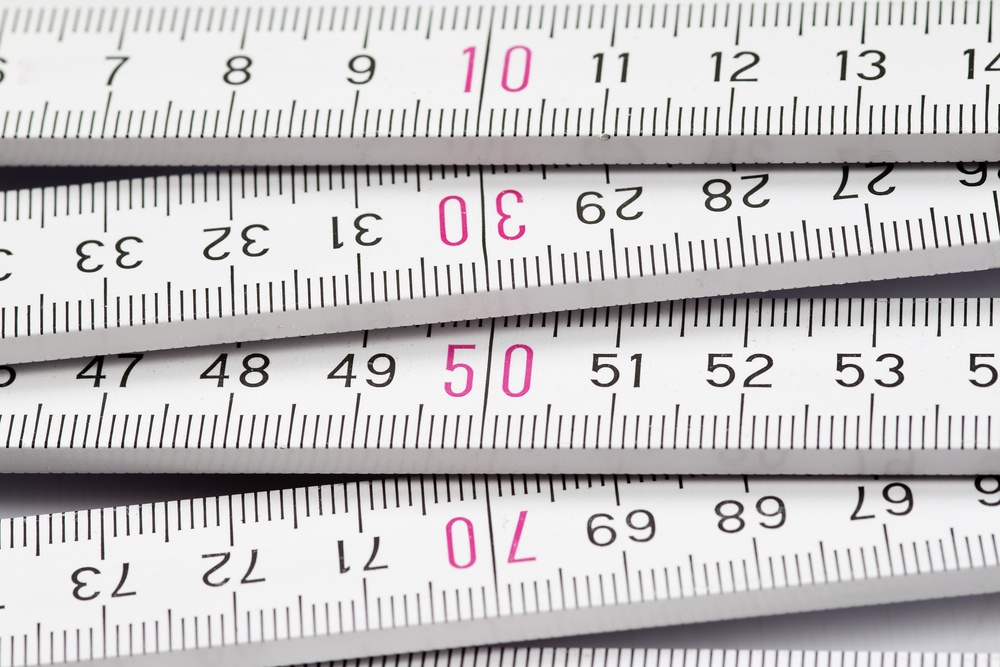
There are a lot of reasons why some people believe measuring cyber risk isn’t possible — from misperceptions about data shortage, to the fallacy about intelligent adversaries, to the inconsistencies that commonly occur when two different people get two different answers when measuring the same risk. I’ve already posted blog articles on the first two in this list, and Douglas Hubbard’s new book (How to Measure Anything in Cybersecurity Risk) covers these kinds of things as well.
In this blog post though, I want to surface an aspect of cyber risk measurement that I’m not sure is commonly recognized — at least explicitly. Specifically, that measuring risk always involves the use of (at least) two models.
Clarity is King
I’ve said it before, and I’ll say it again. You can’t reliably measure what you haven’t clearly defined. We see proof of this repeatedly as we review the risk measurements organizations have been performing and relying on. It’s almost always a bloodbath in terms of their being unable to stand behind the measurements. The fact is, the “wet finger in the air” risk measurement approach so common in our industry doesn’t involve any rigor from a “clarity of what’s being measured” perspective. The results shouldn’t surprise anyone. This is also the primary reason why variability exists between risk analyses performed by two different people. By analyzing risk in financial terms, the FAIR model gives everyone a common language to discuss risk.
But clarity of what?
Risk? Yes. But here’s where we get to the point of this post. It isn’t enough to have the clear model of risk, which is what FAIR provides. You also need clarity regarding the scenario you’re measuring the risk of. That’s the second required model, and it’s the one people new to FAIR struggle with the most. They struggle with it because most of them have never felt compelled to clarify the scope of their wet finger measurements. It can also be a struggle because, well, the risk landscape is complex.
Modeling a scenario involves defining the elements that contribute to a potential loss event — the asset(s) at risk, the threat(s) to those assets, the protective controls that are relevant to the scenario, and the forms of loss that could materialize. The good news is that the more you get used to clearly scoping an analysis, the easier it gets. Even so, anything that can be done to simplify this aspect of the analytic problem is welcome relief.
More than two?
In the first paragraph of this post, I alluded to the fact that more than two models might sometimes be used to measure risk. Without getting too deep into this, I simply want to point out that it can sometimes be useful to explicitly model threat communities (e.g., hacker criminals or rogue insiders) and/or controls as part of a cyber risk analysis. Here again, the FAIR ontology provides a framework for modeling these components of the risk landscape to some degree (e.g., threat event frequency, threat capability, resistive controls, deterrence controls, etc.) but you might find value in other models to evaluate these components (e.g., the STRIDE Threat Model, the Cyber Kill Chain, etc.).
My point is that any complex problem can be parsed into sub-models, which can clarify and ultimately improve measurement. The open-ended question then becomes, how much granularity do you need in an analysis, and how far into the weeds is far enough? After all, diminishing returns applies to risk analysis too.
Unfortunately, there isn’t a hard and fast rule regarding what level of analytic depth is most appropriate for best ROI. To a large degree, it depends on what’s at stake and how much time/resources you have to apply toward analysis. Fortunately, FAIR and other models simplify the scenario modeling component of an analysis, and we're continuing to develop ways to simplify it even more further.