FAIR Cyber Risk Analysis for AI Part 1: Insider Threat and ChatGPT
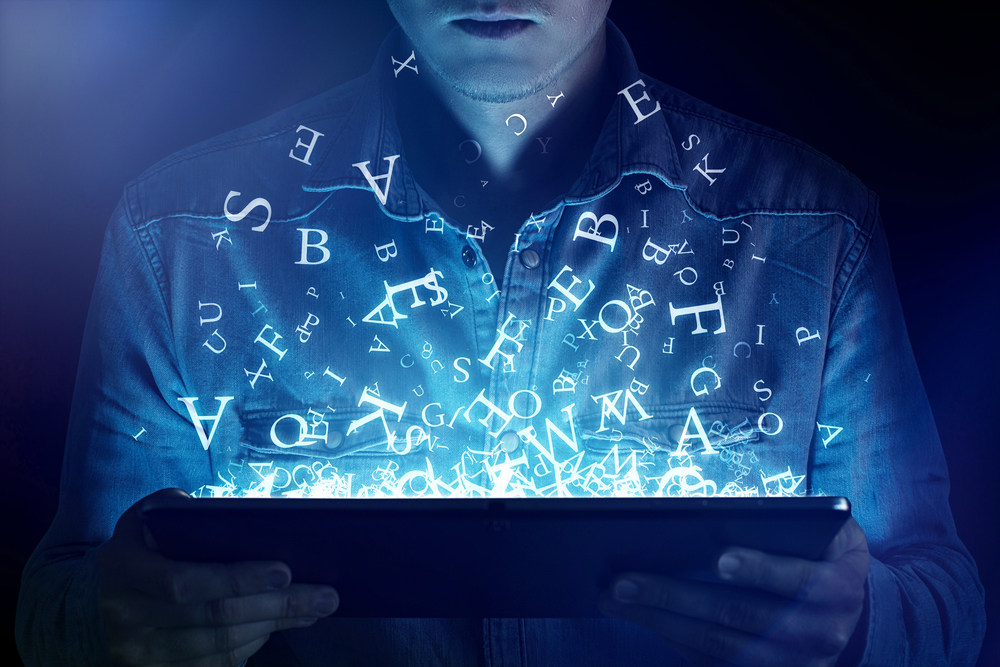
Artificial Intelligence (AI) – once the subject of science fiction narratives – is now a powerful reality shaping the world. In addition to all the exciting possibilities this technology may unlock comes a Pandora's Box of potential drawbacks and risks.
A particularly prominent AI is Large Language Models (LLMs), which can generate uncannily human-like text based on vast amounts of trained data. LLMs' exponential development, application, and user adoption have ushered in a swiftly evolving cybersecurity threat landscape that society must keenly understand and proactively address.
This blog post will explore real-world cyber risk scenarios involving the usage of LLMs and how to approach quantifying potential resulting loss metrics using Factor Analysis of Information Risk (FAIR™).
Michael Smilanich is a Risk Consultant and Kevin Gust a Professional Services Manager for RiskLens.
An Example: Sensitive Data Disclosure at Samsung by Insider via the ChatGPT Artificial Intelligence Chatbot
One such threat involves inadvertently disclosing sensitive information, such as proprietary source code and sensitive corporate data (e.g., board meeting minutes). While this is not a new threat, one of the most popular LLMs, OpenAI's ChatGPT, has opened a new avenue for insiders to disclose sensitive data inadvertently.
To give some context, in March, 2023, The Economist Korea reported three incidents of Samsung employees unintentionally leaking sensitive information to ChatGPT. In two scenarios, separate staff members input confidential source code for error checking and optimization. In another, an employee fed meeting transcripts into the system to summarize the text into minutes.
OpenAI's privacy policy states, "When you use our Services, we may collect Personal Information that is included in the input, file uploads, or feedback that you provide…." Thus, the concern for Samsung and other companies that have experienced similar incidents is that sensitive data input to LLMs gets stored on servers owned by companies operating the services (i.e., OpenAI, Microsoft, Google, and others) and, further, could end up being served to other users as the LLMs continue their machine learning.
Framing a Loss Event Scenario for FAIR Cyber Risk Analysis - Insider Threat and a Large Language Model
To frame this type of incident in a way that allows us to quantify the potential loss exposure, we use FAIR scoping principles to identify a:
- Threat: Non-malicious insider
- Effect: Confidentiality (i.e., data breach)
- Asset: Source code
- Method: Misdelivery (i.e., inadvertently inputting to an LLM)
We approach estimating Threat Event Frequency by determining whether the organization has experienced this threat event in the past, and if it has not, considering how often it might expect to experience this in the future by asking:
- How often are employees using LLMs on corporate devices?
- Why are employees using LLMs (i.e., to accomplish what)?
- Have there been any incidents of insider data disclosure via LLM in the past?
Webinar
Attend a webinar: Quantifying AI Cyber Risk in Financial Terms, hosted by RiskLens, Tuesday, June 20, 2023 at 2 PM EDT.
Next, we estimate Vulnerability (or Susceptibility - see the FAIR definitions) asking:
- Are LLMs blacklisted on corporate devices? As more emerge, how frequently is the blacklist being updated?
- Are adequate data loss prevention (DLP) tools or training in-place to ensure sensitive data is not input into LLMs?
Loss magnitude might be the most difficult to estimate due to the need for historical industry data relative to other commonly quantified cyber risks. However, we could begin to approach estimating loss magnitude by considering the following costs:
- Loss of competitive advantage due to the compromised intellectual property
- Decreased customer trust and customer churn / potential drop in market share
- Incident response
- Reputational damages
- Potential fines or legal expenses
Conclusion: Emerging Cyber Risks in the AI-powered Future
The Samsung incidents were a wake-up call, demonstrating that the AI-powered future holds unfamiliar and complex cyber risk scenarios. These risks necessitate a comprehensive, risk-informed approach to AI governance, and the application of robust risk quantification models like FAIR, powered by a tool like RiskLens, can guide us in navigating this rapidly changing threat landscape. Stay tuned for more posts like this as we strive to stay on top of emerging related cyber risks and discuss approaches to quantifying their impacts.
Read our blog post series on FAIR risk analysis for AI and the new threat landscape