FAIR vs. Proprietary Cyber Risk Analysis Models: What’s the Difference? Jack Jones Explains
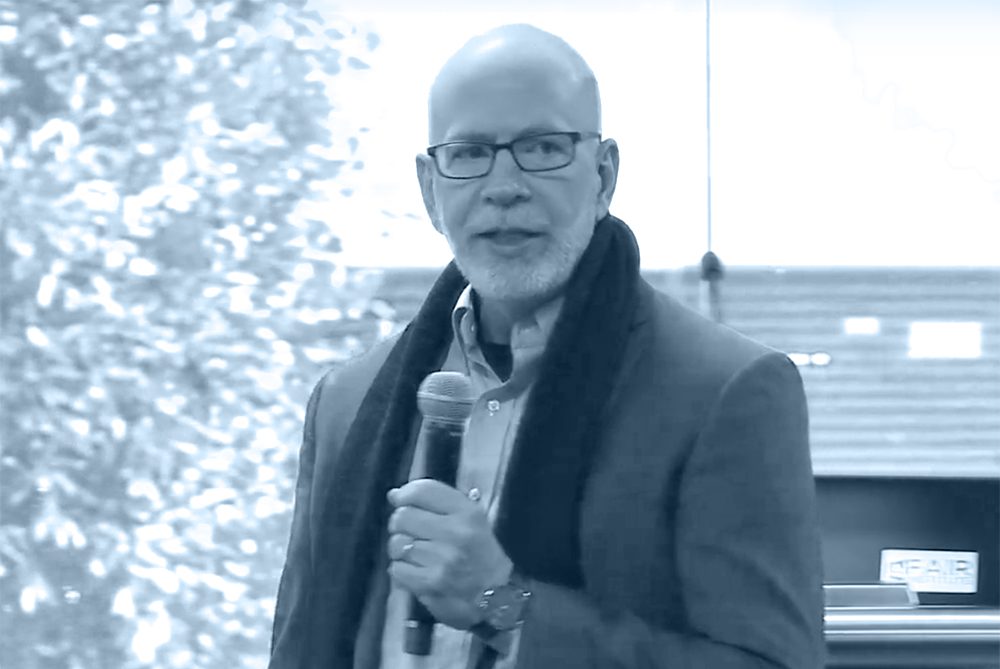
A recent report by an industry research firm stated that, for quantitative cyber risk management, CISOs had to choose among Factor Analysis of Information Risk (FAIR™), proprietary models for risk analysis or a combination of the two. At the FAIR Institute, we think that’s a false equivalency.
FAIR, the international standard for cyber risk quantification, is the only sound choice for CISOs who want to confidently measure and manage cyber risk.
We asked Jack Jones, creator of FAIR, to explain the advantages of FAIR over proprietary information risk models.
Q: Let’s start with the basics. What is a risk model and what should it do?
A: A model is a simplified representation of a more complex reality. It should support analysis by describing how things are related. Common security frameworks are not analytic models because they don’t define relationships, they categorize.
Q: What are the points of difference between FAIR and proprietary models?
A: The biggest difference is that FAIR is open to examination, feedback, and continual improvement whereas proprietary models are not --they could be great or worthless, but users can’t be sure and they can’t push back. For instance, we don’t know what assumptions went into the model.
FAIR is not only an open model, but it also has a book written about it, and there is a professional certification – you know what you are getting. I consider open vs. proprietary risk analysis models to be analogous to proprietary vs. open cryptography; researchers settled long ago that proprietary cryptography is a bad idea because there are so many (and subtle) ways it can go wrong. The very same thing is true for quantitative risk analysis.
FAIR training through the FAIR Institute, advanced and beginner classes online.
Q: How can you tell if a model is broken?
A: Ideally, you test models by doing back-checking; taking risk analysis results and comparing them with loss event data to see how reliably the risk analysis results align with real world experience. Unfortunately, this is extremely difficult to do in cybersecurity. The problem in cybersecurity is two-fold – 1) no single organization has enough loss experience to have statistically meaningful data, and 2) our profession is paranoid about sharing data. This has made it extremely difficult to do meaningful back-checking, although that is beginning to change.
The remaining options for evaluating models boil down to checking the model’s logic, math, and sources of data. For instance, NIST 800-30 violates a fundamental law of probability (for the details, read Jack’s blog post Fixing NIST 800-30).
I also recently encountered a vendor claiming to do FAIR analysis, taking NIST CSF maturity and CVSS scores as data to derive Vulnerability. But NIST CSF has no standardized scoring system and doesn’t take into account dependencies between elements, and CVSS is likewise flawed in a number of important ways. (Learn more: Why NIST 800-30 and CVSS Are Not Enough for Effective Risk Management - by Jack Freund, co-author of the FAIR book).
Using those as a source of data, there’s no way your results will stand up. Also problematic are approaches that apply weighted values to controls. Those weights are incredibly sensitive to context (i.e., they might have a very different weight in a different scenario), and they’re never based on statistical data. Consequently, the results are unreliable. So if your logic, your math, and your sources of data are broken, you don’t need to back-check to know the results aren’t reliable.
Download Understanding Cyber Risk Quantification: A Buyer’s Guide by Jack Jones
Q: What analysis results should you expect from a cyber risk model?
A: It must be scenario-based so you are measuring the frequency and magnitude of loss event scenarios. If a model isn’t scenario-based, I don’t see how the results could be legitimate.
It also should faithfully account for uncertainty using ranges or distributions as inputs and outputs, not as discrete values.
The rationale for the inputs -- why you chose your data range and so forth -- should be open for examination. If analysis was done and there is no rationale, then you should be leery of the output.
Q: You recently introduced the FAIR Controls Analytics Model (FAIR-CAM™). How does that model improve things?
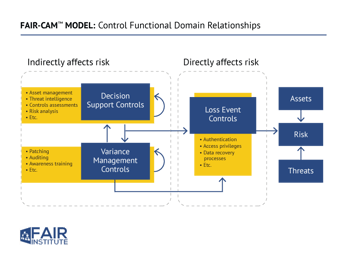

A: To-date, our industry has relied on control frameworks such as ISO, CIS, and NIST CSF or 800-53. These frameworks are very useful as lists of controls that organizations should include in their programs. Unfortunately, those frameworks don’t account for dependencies between controls. This is analogous to practicing medicine and only knowing human anatomy. The human body is a system of highly interdependent parts (physiology), whose relationships are knowable and measurable.
FAIR-CAM describes control physiology – how a system of controls work and interact, as well as what the units of measurement are (vs. ordinal scales). When combined with well-defined control frameworks, FAIR-CAM enables reliable forecasting, measurement, and validation of control efficacy and risk reduction value. This hasn’t been possible before.
Learn more: Watch Out for these 5 ‘Cyber Risk Quantification’ Methods. They Don’t Support Cost-Effective Risk Management